In case anyone missed it: attention on AI’s application to healthcare is apparently at ‘peak hype’. With the volume of healthcare data doubling every 2 to 5 years, it is no surprise that many are using AI to make sense of such vast amounts of data, and development of medical AI technologies is progressing rapidly. At the same time, the COVID-19 pandemic has exposed vulnerabilities in healthcare systems around the world, highlighting the need for technological interventions in healthcare. In line with these trends, the healthcare AI market is expected to grow from US$2 billion in 2018 to US$36 billion by 2025.
The breadth of AI’s application in healthcare is impressive, ranging from diagnostic chat bots to AI robot-assisted surgery. Other examples include AI enhanced microscopes that can more efficiently scan for harmful bacteria in blood samples; efficient and enhanced scanning for abnormalities in radiographic images; and AI algorithm analysis of tone, language and facial expressions to detect mental illness.
But as exciting as the prospects of these AI uses are, exaggerated and unsupported claims about AI’s capabilities in healthcare (such as its superiority over clinicians) threaten to undermine public trust in AI. This is especially important in healthcare, where patients are already in a vulnerable position, the stakes are high and the margin for error is low.
AI’s validity and effectiveness in medicine has been difficult to assess given the lack of standardisation in testing and trial design. In March, a study in the British Medical Journal warned that patients could suffer if public and commercial appetite for healthcare AI outpaces a rigorous evidence base for the effectiveness of AI technologies.
New standards for clinical trials with AI
Randomised controlled trials, or ‘clinical trials’, are globally regarded as the most effective way to verify new treatments and clinical techniques. These trials are used around the world to validate new medical practices, such as drug developments and diagnostic tests, and also underpin the development of health policy.
The CONSORT 2010 (Consolidated Standards of Reporting Trials) Statement and SPIRIT 2013 (Standard Protocol Items: Recommendations for Interventional Trials) Statement are existing guidelines for clinical trials that are endorsed by medical journals around the world. The CONSORT 2010 Statement consists of 25 checklist items regarding the conduct and reporting of clinical trials while the SPIRIT 2013 Statement consists of 33 checklist items concerning the quality of trial protocols.
Last month medical experts behind these leading global standards for randomised controlled trials extended the standards to cover AI technologies (AI Extension). This Extension represents the world’s first official guidelines for clinical trials with AI technologies.
The recent AI Extension recommends that for clinical trials involving AI interventions, 14 new checklist items should be added to the CONSORT 2010 Statement and 15 new checklist items should be added to the SPIRIT 2013 Statement. Among other things, the new checklist items recommend that AI researchers should do the following:
- explain the intended use for the AI intervention in the context of the clinical pathway, including its purpose and its intended users (such as healthcare professionals, patients, public);
- state which version of the AI algorithm was used;
- describe how the input data was acquired and selected for the AI intervention and how poor quality or unavailable input data was assessed and handled;
- specify whether there was human-AI interaction in the handling of the input data, and what level of expertise was required of users;
- specify the output of the AI intervention;
- explain how the AI intervention’s outputs contributed to decision-making or other elements of clinical practice; and
- describe results of any analysis of performance errors and how errors were identified.
In particular, the AI Extension’s working group focused on the safety of AI systems, acknowledging the fact that errors in AI systems are often difficult to detect and explain. The potential for wide scale deployment of AI in healthcare means that a single error could be catastrophic and even fatal. The error analysis checklist item in the AI Extension (CONSORT-AI 19 and SPIRIT-AI 22) was included to emphasise the importance of anticipating and identifying errors in AI systems and their consequences, and having appropriate risk mitigation strategies in place.
But are we behind the game already?
The AI Extension is expected to have a positive effect on the quality and transparency of clinical trials with AI interventions. But given the acceleration of technology with the COVID-19 pandemic, the AI Extension may not go far enough, fast enough.
Importantly, it excludes ‘continuously learning’ AI systems, which have the ability to continually train on new data, meaning that the performance of the AI system changes over time. Continuously learning AI systems are expected to be extremely useful for medical practice as ideally, they will be able to constantly store and acquire data about, for example, a patient’s condition and previous medical history, and use that data to assist clinicians in performing multiple complex tasks such as diagnoses and management decisions.
However, these systems also pose challenges for use in healthcare. Risks such as catastrophic forgetting of data can have severe consequences for the performance of the AI system and ultimately, patients and health outcomes. Given the field of continuously learning AI systems is early in its development and application, the AI Extension’s working group did not consider it appropriate to include considerations for continuously learning systems in the AI Extension.
The omission of these types of systems raises some questions as to the usefulness of the AI Extension in coming years. Despite the challenges in their application, continuously learning AI systems are expected to have significant benefits for medicine in the near-future, and will require rigorous testing and trials to ensure that their deployment is as safe and successful as possible.
The working group did acknowledge that the topic will be monitored and revisited in future iterations of the AI Extension. But given that technological change almost always outpaces regulation, “kicking the can down the road” may not be the best approach here, especially when establishing public trust in use of these AI systems is paramount, and testing standards will likely assist in establishing that trust.
More broadly, if this global approach for medical AI proves successful, the model of sector-based experts developing trial standards for verification of AI technologies could be applicable to other sectors, encouraging an even broader uptake of AI across the economy.
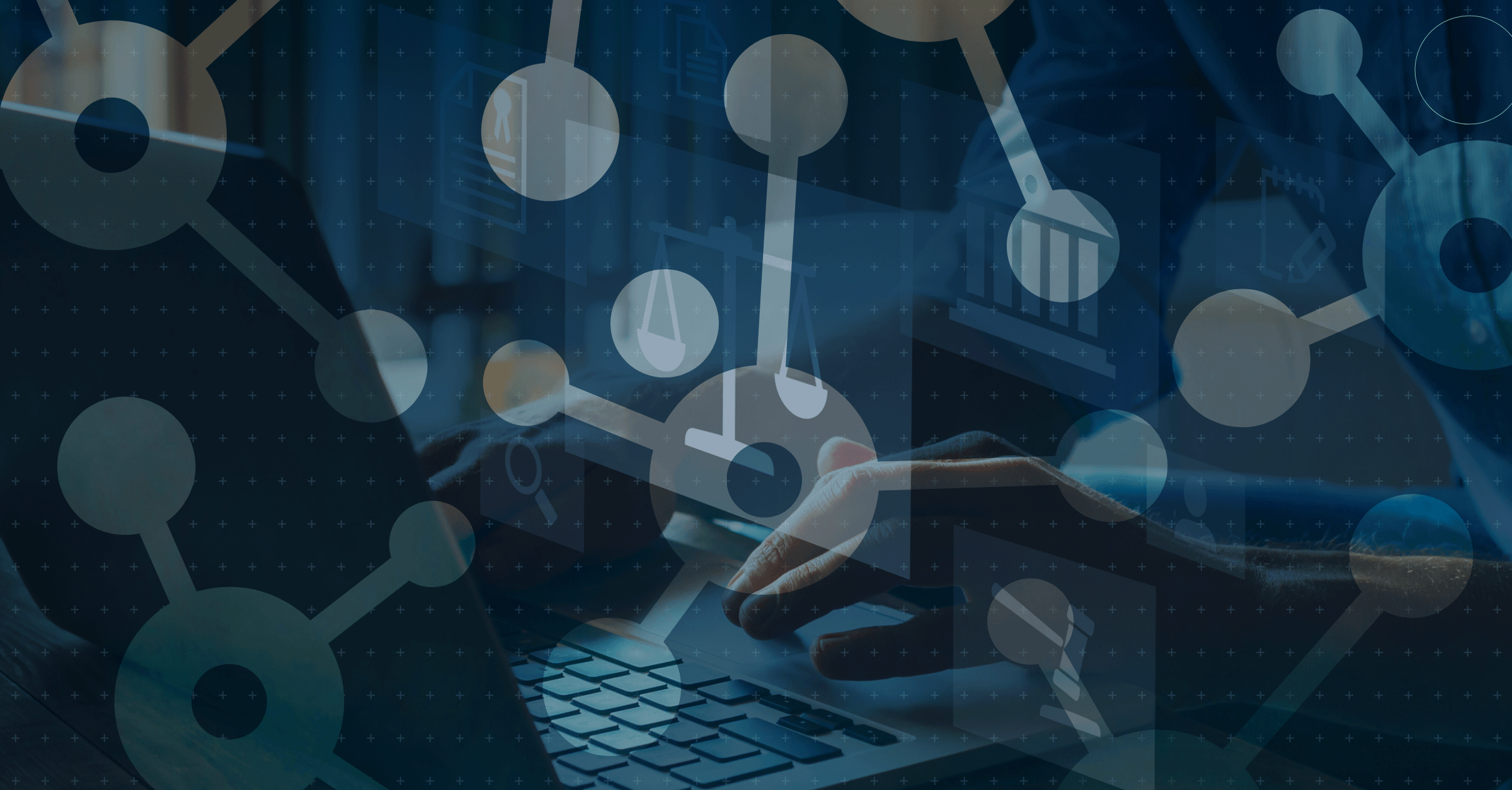
Visit Smart Counsel