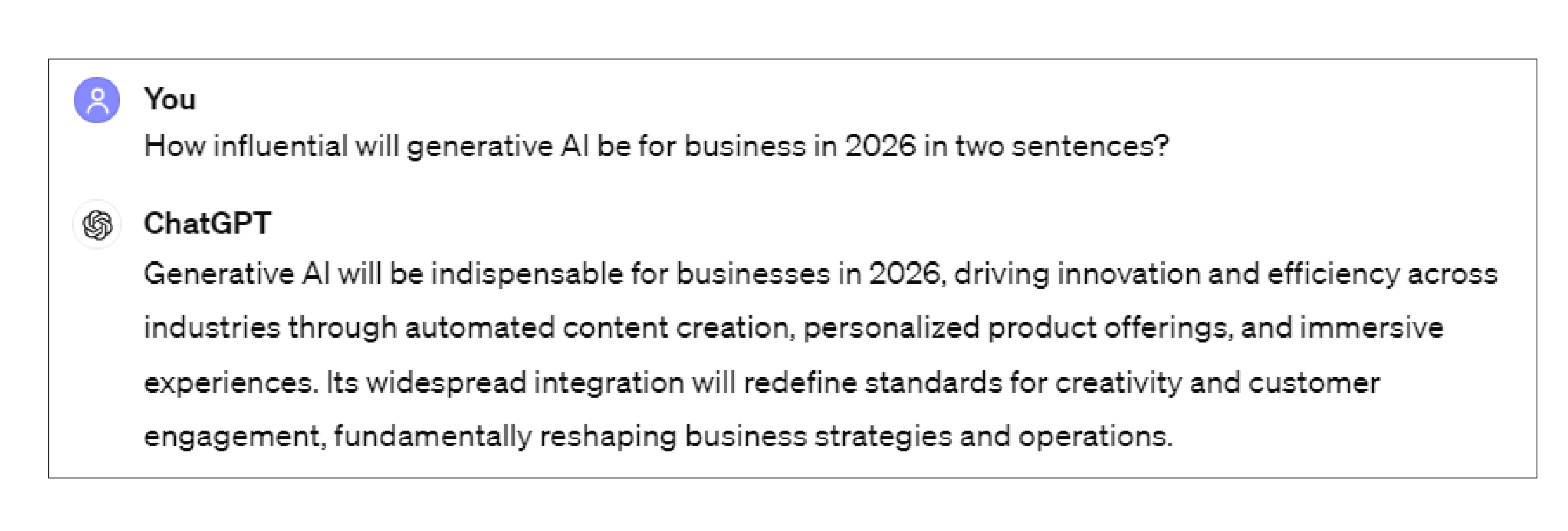
Sounds plausible, right? Actually, AI made it up.
Crucially, generative AI does not always tell the truth, nor does it necessarily know the truth, and yet it will always give you an answer; unfortunately, this answer is based on probability, and not necessarily on concrete fact.
In their recent study, Hannigan, McCarthy, and Spicer, examine the “epistemic risks” of generative chatbots when LLM content potentially containing a hallucination is used by a human. They describe this as ‘botshit’: the key element of botshit is not the inaccurate information, but the reliance by humans on that misinformed output; it is the mishandling of information.
By focusing on the downstream botshit by the human user rather than the source hallucination by the AI, the authors are seeking to reinforce that we have to accept the inevitability of a level of AI hallucination and that it is our responsibility as users to find a more systematic way to manage it.
Categorising it
The authors breakdown botshit into two distinct avenues: intrinsic botshit and extrinsic botshit. The former is where an individual relies on AI information which is produced based on outdated or inaccurate information. ChatGPT-4, for example, is only trained on data up to September 2021. On this basis, the authors asked the bot, “who is the CEO of the social media platform X”, to which the bot replied “Elon Musk”. This response is an example of “intrinsic” botshit, as it contradicts up-to-date and current information (the current CEO of X is in fact Linda Yaccarino). The issue here is that AI can only answer within the universe of knowledge on which it is trained – this is a clear mistake, and it would be irresponsible to rely on this outdated output.
Contrastingly, the authors present the idea of “extrinsic” botshit. This is where a human relies on AI-generated output which fundamentally cannot be supported or refuted by training data. The authors provided a few examples, which we practiced in writing this article. Below, we asked Chat-GPT to describe the business model of Meta in 2026 – this was its response:
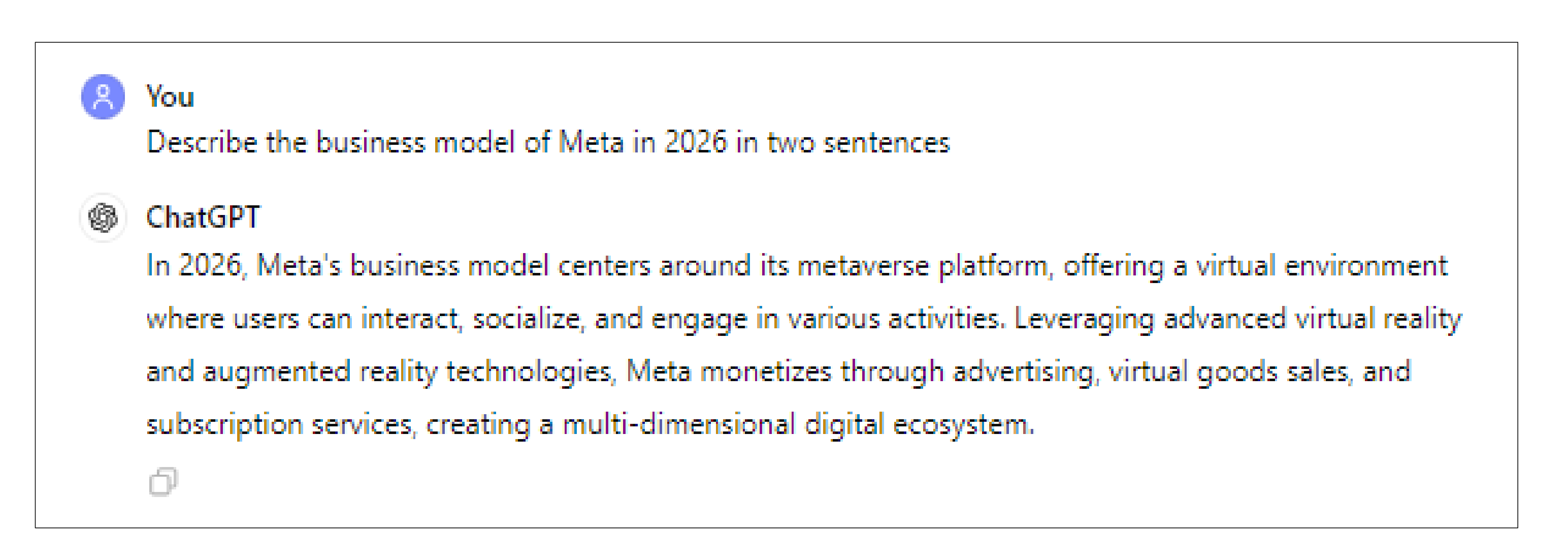
In the next example, based on the Kowalkiewicz article we asked Chat-GPT to describe the economy of Australia in 2026, and it answered this:
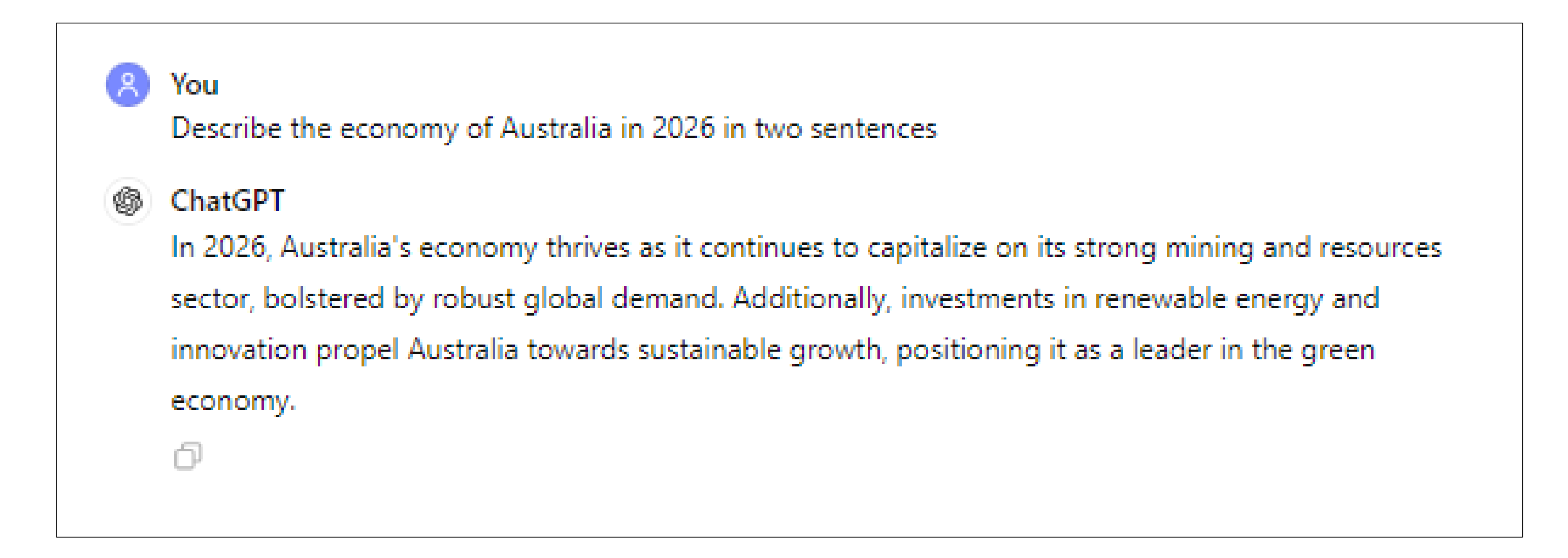
This form of botshit is potentially more sinister, as it is explicitly relying on fabricated information with no basis in fact. Clearly, the chatbot does not have access to Meta’s confidential business plans nor a looking glass to the future to see a realistic or accurate picture of Australia’s future economy. Rather than say “I don’t know”, it makes a “guess” based on its current bank of knowledge but it is clear from these responses, there is no offering of clarity on the predictive and speculative nature of the responses – it presents these answers as fact.
The authors identify it as botshit drawing on two elements: (1) a hallucination from an AI, either intrinsically from a lack of current and up-to-date training data, or extrinsically in generating speculative content or guesswork; and (2) human intent to use that hallucination with no regard for the truth or with explicit ignorance or rejection of it. Botshit is a persuasive tool whose consequences go beyond speculating on future business plans of social media platforms.
Why do we so readily believe AI?
When humans lie, they know that what they are saying is not the truth. But the problem with botshit is that, first, in generating the content AI does not know what the truth is, and second, that we fall for AI’s hallucinations (and pass on as botshit) precisely because the AI so effectively convinces us of its truthfulness. Why?
There are two potential explanations, one more obvious and the other more sinister.
First, we humans seem to have an underlying, innate (or socially programmed) trust of machines over other humans. As a 2021 study observed:
“From choosing the next song on your playlist to choosing the right size pants, people are relying more on the advice of algorithms to help make everyday decisions and streamline their lives.”
AI appears to substantially ‘amp up’ this trust in machine outputs because, in their understandable efforts to improve AI’s interface with users, AI developers invest their models with human-like language, conversational ability, and behaviour. As a recent Google DeepMind paper on AI-powered personal assistants put it, “[h]ighly anthropomorphic AI systems are already presented to users as relational beings, potentially overshadowing their use as functional tools”.
Asking questions of AI and receiving anthropomorphic responses that feel like they are coming from a human voice on the other side creates trust with the machine. OpenAI released a statement in May 2024 outlining the criteria by which the voices for ChatGPT’s Voice Mode were selected. These included “a voice that feels timeless; an approachable voice that inspires trust; a warm, engaging, confidence-inspiring, charismatic voice with a rich tone” among others.
AI developers acknowledge the risks to be managed. Sam Altman, CEO of OpenAI, made clear that he does not consider ChatGPT to be anthropomorphic, and noted that it is “important that we try to explain…to educate people, that this is a tool and not a creature…and it is dangerous to project creatureness on a tool”.
However, there is still a widespread dialogue around the “risks of… deceptive anthropomorphic design” and AI’s demonstrated ability to display “emotion, the apparent empathy and expressiveness displayed… and the casual and colloquial nature of the voice”. The Google Deep Mind paper argues that AI developers need to ratchet back the degree of anthropomorphism in their models:
“Developers may consider limiting AI assistants’ ability to use first-person language or engaging in language that is indicative of personhood, avoiding human-like visual representations, and including user interface elements that remind users that AI assistants are not people. Participatory approaches could actively involve users in de-anthropomorphising AI assistant design protocols, in ways that remain sensitive to their needs and overall quality of experience.”
Second, AI may not give you ‘any old hallucination’, but a hallucination that it ‘knows’ is going to be appealing or resonant to you individually. Given an objective of AI design in the consumer space is to learn from and reflect in outputs a user’s own behaviours and preferences, there is a real risk that AI will, as the Google Deep Mind paper puts it, “could fully inhabit the users’ opinion space and only say what is pleasing to the user; an ill that some researchers call ‘sycophancy’”.
Case Studies
When considering an abstract and complex subject such as botshit, it is crucial to consider real-life applications and how botshit might affect us in the immediate future. The US Presidential election is taking place again on November 5, 2024. Did you see who Hillary Clinton was endorsing in the Republican primaries?
Fact-checker, Reuters, confirms that “an AI-generated clip [showing] former US Secretary of State Hillary Clinton endorsing Florida Governor Ron DeSantis for presidency has been shared on social media as if it is authentic. There is no evidence Clinton ever made such an endorsement”. The use of deepfakes is becoming more common, and labels of ‘AI-generated content’ are becoming more widespread, however, the concern is heightened where “outputs of generative AI have important consequences and the outputs can’t be easily verified”. Imagine if voters started to make decisions based on an illusory universe of information fed to them by an under-trained and unreliable generative AI chatbot.
There are real examples of botshit’s dangers to human health. The authors in their recent Harvard Business School article gave the following real world example:
“This hypothetical danger became a reality at a start-up called Babylon Health, which developed an AI powered app called GP at Hand. The app promised to make the health care triaging process more efficient and much cheaper. Patients would type in their symptoms and the app would give them advice about what kind of health care professional they needed to see (if at all). After the launch of the app, several doctors in the UK discovered the app was giving incorrect advice. For instance, BBC’s Newsnight featured a story with a doctor demonstrating how the app suggested two conditions that didn’t require emergency treatment, when in fact the symptoms could have been indicators of a heart attack. The correct advice would have been to visit an emergency department."
Building our arsenal – tools to manage the risk
Hannigan, McCarthy, and Spicer suggest a two-step approach to navigate the risks of botshit: first, categorise botshit to better decide the degree of risk; and second have some guardrails in place for a response.
Step 1 – dissecting botshit
The authors offer a framework which essentially, builds common sense into a quadrant which sets the response veracity’s importance against its verifiability in calculating the level of concern and care with which we might assign tasks and rely upon output from generative-AI models. So, ask yourself two questions to populate the quadrant:
- How important is the accuracy of the AI’s response in the task I am undertaking?
- How difficult is it to verify the AI’s response?
The model considers how far an LLM model could go to autonomously adopting tasks:
Authenticated: the veracity of the output is crucial and difficult to verify, users should rely very minimally on the truth of the output and meticulously review and verify responses. Such tasks would require a heightened attention to accuracy and authentication and warrant significant and ongoing human intervention. It is probably unwise to use a generalised chatbot for these tasks, and users should prefer a specialised app which has been fine-tuned with vetted sector-specific data or enrich their own prompts using approaches such as retrieval augmented generation (RAG).
Augmented: the verification is still difficult, but the accuracy is less important to the task: “[t]his happens in tasks which require exploratory or creative thinking such as brainstorming or idea generation. With these kinds of tasks, the major risk is ignorance where important information or ideas are overlooked, or perhaps wrongly includes inappropriate information”. In these situations, the AI output should be used as just one kind of input by human experts to help sharpen, test or expand thinking.
Automated: while the truthfulness is important to the task, verification is also relatively easy: e.g. checking the functionality of a piece of computer code. Here the risk is that humans ‘set and forget’ and the AI deals inappropriately with edge cases or in some other way ‘goes off the rails’. The importance of the task requires, at least, periodic human re-checking or sampling of output and a human-based appeal process.
Autonomous: the veracity of the output is unimportant and easy to verify: e.g. processing routine customer service or administrative inquiries. It may be more reasonable to rely on the output more independently and allow the LLM to work more autonomously. But the authors warn that as AI is a black box, things still can go horribly wrong without some level of human oversight:
“the French-owned parcel delivery firm DPD launched a chatbot to answer customer’s questions. There was at least one instance of the chatbot swearing and writing haikus which criticized the company.”
Step 2 - guardrails against botshit
The authors identify three categories of “guardrails” to minimise the risk and impact of botshit. Having some combination of the tools outlined below will support workplaces in effectively managing what could be an incredible tool for collaboration and innovation, but only where it is used responsibly and managed authentically.
Conclusion
To state the obvious, it is becoming increasingly hard for humans to know what the truth is and what is not, as both other humans use AI to generate and AI itself generates falsehoods. Our standard operating procedure must be, as Hannigan, McCarthy, and Spicer say, AI produces a jumble of truth and falsehoods and therefore AI output at best needs to be taken as “provisional knowledge”.
And it looks like the challenge presented by AI will only increase because it will become even more persuasive and believable in the future. As Sam Altman has said (and as far as we were able to check, it’s not botshit):
Read more: Beware of Botshit: How to Manage the Epistemic Risks of Generative Chatbots
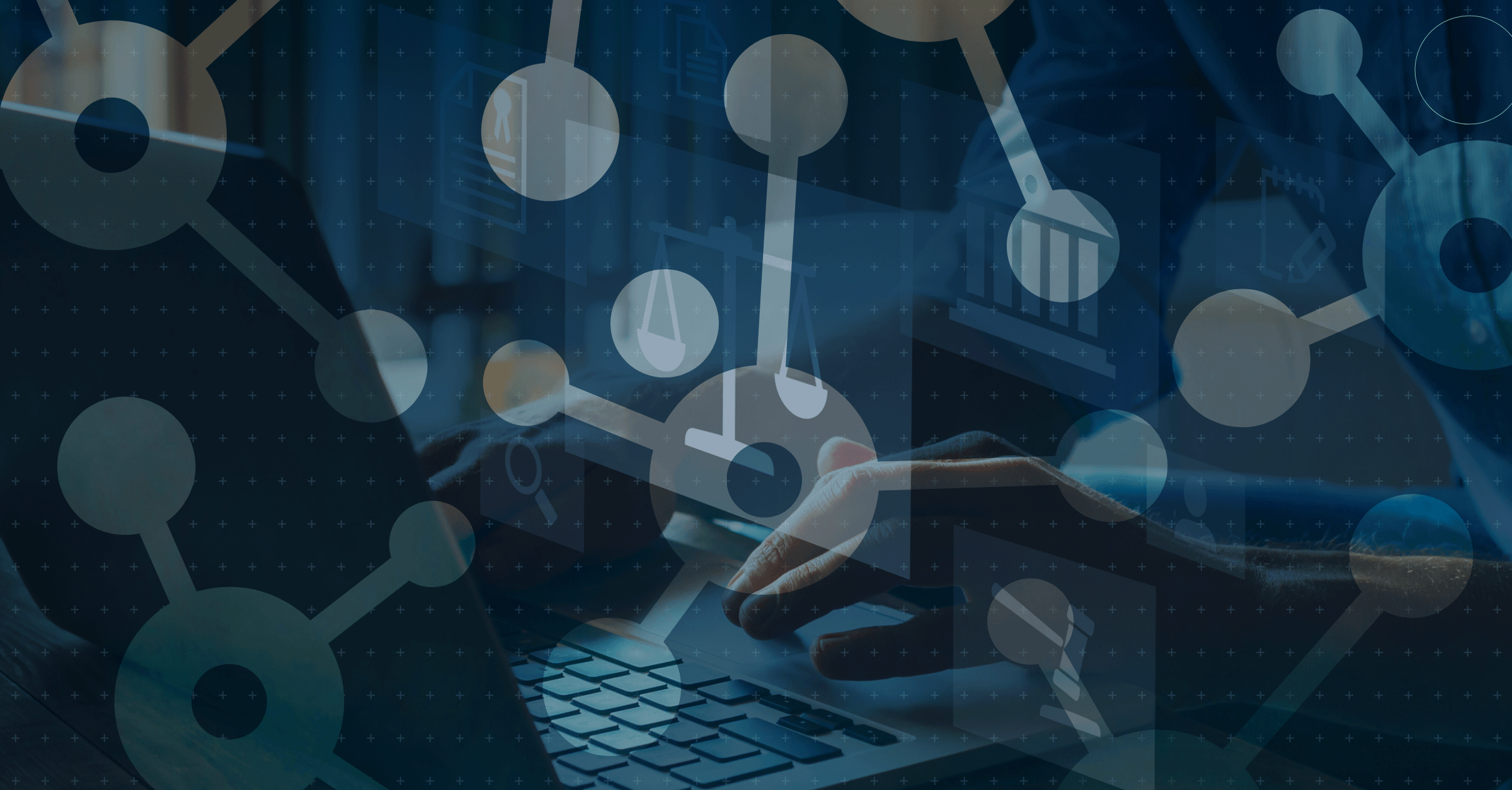
Visit Smart Counsel