The UK has a 10 year strategy to become an AI superpower. A recent frank assessment by the Alan Turing Institute and Technopolis, based on surveys and interviews across AI researchers and institutions, found the UK won’t get there without a substantial investment in ‘digital research infrastructure’ (DRI).
There are lessons here for policymakers in other countries who recognise that AI leadership is necessary for economic and social development, even with all AI’s hazards.
What is digital research infrastructure?
The DRI report defines DRI as the technological, data and human resources and skills with which a country innovates in AI, including “large scale compute facilities; data storage facilities, repositories, stewardship and security; software and shared code libraries; mechanisms for access, such as networks and user authentication systems; and the people, users, and experts who develop and maintain these resources”.
The Canadian Government is blunter in its description of DRI and its importance :
“Digital research infrastructure is the collection of tools and services that allow researchers to turn big data into scientific breakthroughs. As the global innovation race speeds up, only the countries that have world-class digital research infrastructure in place will be able to stay competitive.”
How big is your computer?
AI needs ingests oceans of data and needs massive computing power to do so. In particular, the computing power from Graphics Processing Units (GPUs), which have specialised processing units with enhanced mathematical computation capability, are advantageous for AI workloads. The highest level of computing currently achieved is called exascale which, at 1,000,000,000,000,000,000 operations per second will be able to quickly analyze massive volumes of data and more realistically simulate the complex processes and relationships behind many of the fundamental forces of the universe.
The DRI Report considers that central to a country’s DRI is access to large scale, state-of-the-art computing power, but as the report notes, “it is often not considered holistically in policy making.”
There are some expected stand-outs:
In the United States, Oak Ridge National Laboratory’s Frontier supercomputer boasts was the first system to achieve exascale, currently ranks first on the TOP500 list of the biggest global computers, and is more powerful than the following seven TOP500 systems combined. With a $500 million spend, the US will soon commission the Aurora exascale computer which will have double Frontier’s computing capacity. Oak Ridge’s supercomputers supported AI which rapidly developed an efficient COVID drug discovery process, winning the supercomputing world’s equivalent of a Nobel prize.
The European Union will soon commission the European High Performance Computing Joint Undertaking (EuroHPC JU) with an exascale computer in Germany and four new mid-range (petascale and pre-exascale) supercomputers in Greece, Hungary, Ireland, and Poland.
China has the highest number of supercomputers in the world (173 of the TOP500), which translates to 12 percent share of the list’s aggregated performance.
The DRI found two shortfalls in the UK’s computing resources for AI. First, the UK does not have a national computer (Tier 1) capability for researchers wishing to use AI tools and techniques, with only 11 computers in the TOP500 list accounting for only 1.2% of supercomputer capacity. Worse still, the UK’s national supercomputing service ARCHER2 does not include the accelerator hardware required for most AI approaches.
Second, in the academic equivalent of “tinkering in their garden sheds”, UK AI researchers typically use their research group’s, lab’s or institutional own computer as their primary resource. While having the benefits of easier and more convenient access, these local computing systems are underpowered. As a result, 50% of survey respondents stated that compute provision did not align with their requirements.
Even more strikingly, around half of respondents were currently using commercial cloud (e.g. Amazon Web Services, Microsoft Azure, Google Cloud Platform) for their AI-related work. Many interviewees predicted that the use of cloud will continue to increase in future, as it addressed researchers’ needs for flexible, convenient access to compute, without lengthy proposal processes. Interviewees also indicated that cloud was particularly useful for prototyping and demonstrations, meeting spikes in compute demand (i.e. cloud bursting), or to meet specific hardware or software requirements. However, the DRI report cautioned that “greater reliance on public cloud provision could create additional challenges for researchers around data security, path-dependency and increasing costs.”
The pressure on UK computing resources will accelerate, with most researchers predicting their demand for computing capacity would double in the next 5 years.
The DRI Report called for the UK to do more with the tier 2 resources it has by better co-ordinating their use, access mechanisms and permission processes and data inputs.
My data, Your data
The DRI Report observes that ‘[t]he lack of availability of data for AI is a common problem across research and innovation communities and presents a barrier to almost all AI-related research fields.”
The DRI Report found that data collection and storage was mainly an ad hoc DIY exercise by individual researchers. The majority of survey respondents source their data from a combination of open / freely available data sources (77%), academic collaborators (69%), or their own sources (61%). The majority then stored this data on institutional / organisational services (89%) or on their individual computers (70%).
45% of survey respondents said that using data from multiple sources - usually considered essential to avoid biases in AI - was a significant or moderate barrier to AI research. Around a third of survey respondents also indicated that the time required to adapt existing data for AI purposes was an important barrier in relation to the availability and suitability of data for AI. Many researchers pointed to the multiple, bureaucratic processes to be completed to secure access permissions, and then for linking data sets.
These problems are only going to get worse, with the DRI Report concluding that the amount of data that researchers are working with is expected to increase tenfold over the next five years.
The DRI Report made two recommendations:
specific funding and projects to support research communities to develop data management standards and communities of practice in research fields where data-intensive research is emergent.
centralised organisations within key research fields that are responsible for collating, standardising and / or integrating datasets from disparate sources: “Such organisations can also play a key role in reviewing and critically evaluating datasets (e.g. for bias, gaps, or more inherent structural issues), which is especially valuable for researchers with less experience working with large data sets.”
South Korea has pushed similar strategies to build a national ‘ data dam ’ to collect data generated through public and private networks and to standardise, process, and utilize the data to create smarter AI . There is even a "crowd sourcing" option to allow ordinary citizens to contribute.
The people part of AI
Survey respondents indicated that, after access to computing systems with GPUs, the three highest priority areas to meet their current and future needs were many more research software engineers (62%), training for researchers (61%), and funding for general technical support services (61%).
The DRI Report noted the importance of the behind-the-scenes tech heads: “researchers often need expert support to help them with adopting AI tools and libraries and best development practices, as well as exploring and exploiting DRI for their research.” However, technology professionals within universities are often relatively small in number and have to work to support the breadth of needs across the university. Universities struggle to match the salaries paid by Big Tech.
As AI can operate across or be applied in many sectors, there is an increasing need for multi-disciplinary approaches in the research and development phase of AI or in the use of AI for research. Universities are typically not good at working on an interdisciplinary basis. The DRI Report concludes:
“To enable this, there is a need for cross-domain specialists with expertise in AI who are also able to work collaboratively with domain specific researchers to support the application of AI tools to their workflows. As it stands, many research communities only have a limited number of individuals who can “translate” the different needs and requirements from an AI perspective and a domain specific perspective. This is especially valuable in research fields without a strong history of data intensive research such as in the arts and humanities.”
Researchers who use AI in the course of their research do not need to become AI experts themselves, but they need a level specialist training on how AI works. Even amongst researchers currently developing or applying AI, 37% of respondents reported they currently had poor or very poor skills in organising and structuring data and/or code.
Take-outs for building the AI future
The DRI Report concludes that “an integrated and holistic programme of support for compute capacity, data access, and people and skills” is needed if the UK wants to meet its ambition of being an AI superpower.
That easier said than done - especially in a smaller economy like Australia with more limited resources. Australia has only 5 computers in the TOP500 list, and only 1 in the top 100.
Yet size has not been a barrier to other countries investing in supercomputing resources and DRI generally. While a national exascale computer may be beyond Canada’s capacities, its Digital Research Alliance coordinates access to its five major supercomputers each offering between two and six petaflops, operated by regional partners across the country. In addition, Canada has four national AI institutes, one of which, the Vector Institute, operates its own AI computing infrastructure, which provides 12.5 petaflops performance.
Read more : Review of Digital Research Infrastructure Requirements for AI
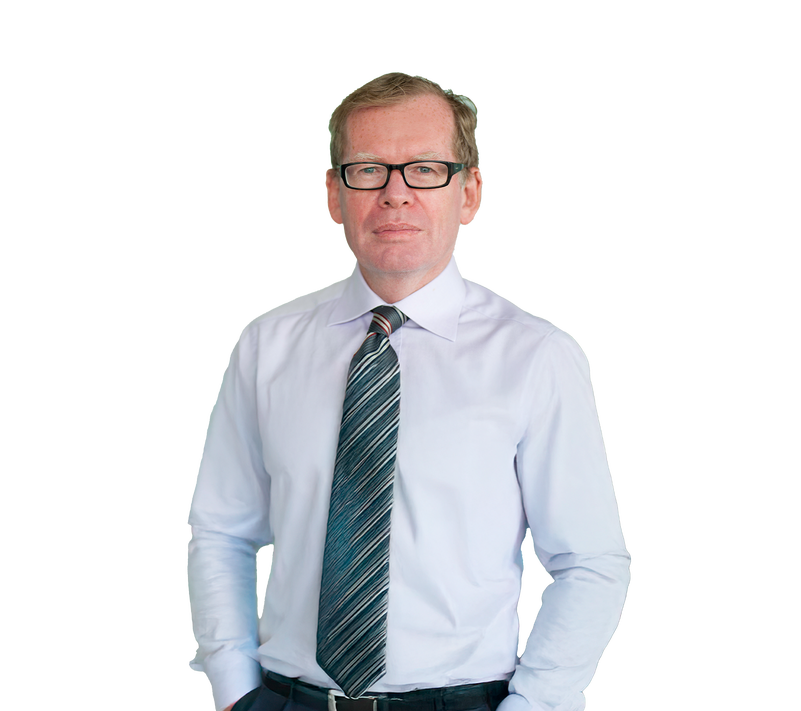
Peter Waters
Consultant