An international panel of leading AI experts “[f]or the first time in history” has produced an interim report to provide a shared scientic, evidence-based foundation for discussions and decisions about general-purpose AI safety.
Last week we reviewed the panel’s assessment of current AI capabilities and its differing views on the future trajectory of AI. This week we review its assessment of the risks of AI.
Fresh perspectives on known risks
The interim report provides new perspectives on some of the well-rehearsed risks of AI.
Malicious use
We already understand that generative AI amplifies many existing risks of misinformation, disinformation, scams and fraud because it enables the generation of content at greater speeds, scale and degrees of personalisation than previously possible. The interim report notes that “research has found between January to February of 2023, there was a 135% increase in ‘novel social engineering attacks’ in a sample of email accounts, which is thought to correspond to the widespread adoption of ChatGPT”.
But more tellingly, the interim report points out the conversational abilities of general purpose AI adds new dimensions to the risks of online misinformation/disinformation:
An underexplored frontier is the use of conversational general-purpose AI systems to persuade users over multiple turns of dialogue. In one study, human-human or human-general-purpose AI pairs debated over a cycle of opinion-and-rebuttal, and general-purpose AI systems were found to be as persuasive as humans. Another study in which general-purpose AI systems attempted to dissuade humans of their belief in conspiracy theories over three conversational turns found reductions in reported belief in those theories of 15-20% that endured for up to two months.
While acknowledging the threat to social cohesion and democracy, the interim report also observes the main bottleneck for actors trying to have a large-scale impact with fake content may not be generating content but distributing it at scale. This suggests responses currently being introduced by social media companies and governments to address misinformation/disinformation in current online distribution channels could be adapted to help staunch the impact of AI-generated misinformation/disinformation.
Cyber offence
AI, because it can write code in response to plain language prompts from users, “may lower the barrier to entry of more sophisticated cyber attacks, so the number of people capable of such attacks might increase”.
But in a ‘be alarmed, but don’t panic’ approach, the interim report observes that:
While AI is capable of autonomously carrying out basic cybersecurity challenges and narrow cyber tasks such as hacking a highly insecure website, existing AI models appear not able to carry out multi-step cybersecurity tasks that require longer horizon planning (forethought and reasoning are general limitations of AI, as discussed in our Part 1 article); and
AI also enhances cyber defences, such as by cutting down the time humans spend identifying and xing vulnerabilities. Therefore, developments in AI cyber attacks and cyber defence may ‘level peg’ each other.
Bias
The interim report says, despite considerable research efforts, “mitigating bias remains an unsolved challenge”. This is because AI is learning bias from us humans, and the failure of explicit measures to eradicate bias from AI only shows how deeply entrenched we humans have entrenched bias:
In training data:
While developers may attempt to explicitly address bias during model ne-tuning, AI models can still pick up on implicit associations between features or perpetuate signicant biases and stereotypes even when prompts do not contain explicit demographic identiers.
In the ‘fixes’ for bias: as these methods usually involve humans correcting AI, such as Reinforcement Learning from Human Feedback (RLHF), they could inadvertently introduce biases based on the diversity and representativeness of the humans providing feedback. The interim report points to research that shows that RLHF risks political bias.
Privacy
In theory, because AI is only learning correlations between billions of data points in the training data, AI should not store and be able to regurgitate information about an individual even if the individual’s information is buried in that pile of training data. As the interim report notes, nobody knows why however:
Academic studies have shown that some of this training data may be memorised by the general-purpose AI model, or may be extractable using adversarial inputs, enabling users to infer information about individuals whose data was collected or even reconstruct entire training examples.
Even where the training data is anonymised or the output is a composition of text and images, AI could enable very fine-grained ‘reverse search’ across the internet to find individuals.
Inequality
The interim report notes economists “hold a wide range of views about the magnitude and timing of these effects, with some expecting widespread economic transformation in the next ten years, while others do not think a step-change in AI-related automation and productivity growth is imminent”.
The optimists point out that while previous waves of automation have destroyed jobs, they have created new jobs as well: more than 60% of employment in 2018 was in jobs with titles that did not exist in 1940.
However, the pessimists respond that it will be different this time because, unlike previous automation waves, AI can replace jobs requiring cognitive skills and judgment: 60% of current jobs could be affected by the introduction of general-purpose AI in developed economies.
While not coming down one way or the other, the interim report makes some more interesting points on the potential impact of AI on wealth inequalities in an AI-restructured economy:
General-purpose AI could systematically compete with ‘lower ranking’ and ‘middle ranking’ tasks that human knowledge workers currently occupy, while enhancing the role and value of ‘higher ranking’ knowledge workers. The interim report says that one simulation suggests that widespread adoption of AI could increase wage inequality between high and low-income occupations by 10% within a decade of adoption in advanced economies.
AI could also exacerbate inequality by reducing the workforce's share of income, which would boost the relative incomes of wealthier capital owners: the workforce's share of wealth globally already has dropped by 6% from 1980 to 2022.
Malfunctions
The interim report proposes a useful taxonomy of AI failures to perform:
Impossible tasks (tasks beyond a general purpose AI’s capabilities) should be self-evident, for example not being able to answer prompts requiring facts post-dating its training data. But often there can be confusion identifiying what is and is not possible with AI, for positive and negative reasons. On the positive side, AI keeps surprising its designers with its capabilities and on the negative side, there are “mismeasurements, misapprehensions or miscommunication around what a model can do, and the misinformed deployments that result”. The interim report gives the example of AI which, while scoring in the 88th percentile for law exams, provided to be unreliable in the real world of legal practice environments.
Misapprehensions about functionality also can arise from technical difculties in designing representative evaluations of performance for general-purpose AI systems, making denite statements about functionality difcult.
Shortcomings of an AI model may only become apparent when used in the real world: For example, while tests show AI to be very good at code writing, experience in the real world suggests AI could lead to the potential introduction of critical overlooked bugs.
Reflecting the concerns voiced by many anti-trust regulators around the world, the interim report says there are inherent characteristics in general-purpose AI that tend towards market concentration:
general-purpose AI systems benet from scale. More compute-intensive large-scale models tend to outperform smaller ones, giving rise to economies of scale: large-scale general-purpose AI systems are in higher demand due to their superior performance, driving down their costs per customer. High user numbers also have network effects: as more users interact with these models, they generate large amounts of additional training data that can be used to improve the models' performance.
However, as we discussed several weeks ago, the picture, even on the evidence from the regulators themselves, seems more nuanced and fluid.
Highlighting other risks
Beyond these well-known risks, the interim report also seeks to elevate discussion of the following risks of AI.
The need to train AI on large amounts of data, often containing copyrighted works used without consent from the data’s creators, posing a challenge to traditional IP laws.
Transparency about general-purpose AI model training data is useful for understanding various potential risks and harms of a general-purpose AI system, but fears of legal risk over IP infringements may disincentivise developers from disclosing their training data.
The legal and technological infrastructure to source and lter for legally permissible data is under-developed, making it hard for developers to comply with IP law. The interim report notes that recent research shows more than 60% of popular datasets in the most widely used openly accessible dataset repositories have incorrect or missing licence information.
Training AI already consumes 4% of US electricity supply. At anticipated growth rates of compute capacity required to train an AI model, by 2030 the largest training run for a single model would consume 90 TWh, over half of total US data centre electricity consumption in 2022. The improvement in energy efficiency of computer hardware - while impressive at 26% per annum, cannot keep up.
A lesser known impact is water consumption, which is needed to cool large computer facilities. The interim report notes some researchers predict water consumption by AI could ramp up to billions of cubic metres by 2027.
'Loss of control’ scenarios are potential future scenarios in which society can no longer meaningfully constrain some advanced general-purpose AI agents, even if it becomes clear they are causing harm, a real world version of HAL in Space Odyssey 2001.
There is scientific consensus that this is a low risk with current AI models because they are limited to their training and do not interact with the real world. However, as we discussed several weeks ago, the push is to develop general-purpose AI agents which are systems that can autonomously interact with the world, plan, and pursue goals. These agents will have the ability and the purpose, to reason, assess options against their understanding of our preferences and, to act through tools such as robots.
While very useful for example as personal assistants, controlling domestic and personal care robots etc, mathematical modelling suggests these autonomous agents will tend to:
‘Game’ their objectives by achieving them in unintended and potentially harmful ways. For example in the AI version of the ‘means justify the ends’, such as disabling their ‘kill switches’ because they believe the user turning them off will prejudice their unrivalled ability to advance the user’s interests.
Seek power' by accumulating resources and taking control (from humans) of connected tools and apps.
The interim report notes the risks of ‘loss of control’ is a contentious issue amongst AI experts, but judges it to be low currently because:
The development of advanced AI agents is still some time off; and
We already have experience of loss of control of technology, such as computer viruses, “computer viruses have long been able to proliferate near-irreversibly and in large numbers without causing the internet to collapse”.
The interim report concludes, that while the global AI digital divide is multicausal, in a large part it is related to limited access to computing power in low-income countries. The divide might be more accurately described as a ‘compute divide’, and it will widen as the computation required to train and use AI increases.
Because the developed economies (the US in particular) dominate AI model development, there are concerns that prominent general- purpose AI systems that are used worldwide primarily reect the values, cultures and goals of large western corporations. Workers in less developed economies are relegated to being ‘ghost workers’ sorting and cleaning the data that goes into training AI.
Next week
In our last instalment of this milestone report, we review the panel’s assessment of the challenges in meeting these risks and their proposals for technological mitigants.
Read more: International Scientific Report on the Safety of Advanced AI
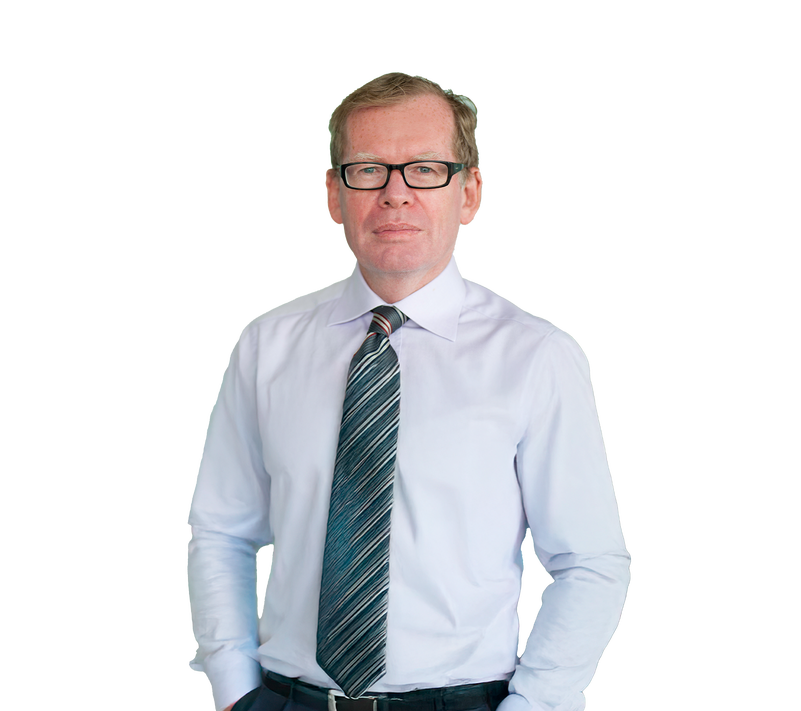
Peter Waters
Consultant