Last week we discussed botshit and how to manage it. This week we review a proposal from Oxford University researchers (Wachter, Mittelstadt and Russell) to fundamentally shift AI developer incentives by imposing a legal obligation to design truth-telling AI.
Truth as an accident of probability
AI, by dint of its design, is not only unconcerned about, but is also unable to determine the truthfulness of its output, because AI produces answers based on predictions. The result is that, as the Oxford researchers say, large language models are only incidental truth-tellers. Outputs will often be correct or at least based on factual information due to reliable information appearing frequently in the model’s training data, but equally the more that a falsehood has been written on the Internet, the more likely it is to be chosen in a response called common token bias.
The Oxford researchers argue this inbuilt risk of AI generating inaccurate responses is compounded because AI developers built their models to strain to be helpful to users:
“Unlike human speakers, LLMs do not have any internal conceptions of expertise or confidence, instead always ‘doing their best’ to be helpful and persuasively respond to the prompt posed. They are designed to participate in natural language conversations with people and offer answers that are convincing and feel helpful, regardless of the truth of the matter at hand.”
While the tendency of humans to anthropomorphize machines is well established, this can be reinforced by the increasing ‘humanness’ of AI interfaces. Open AI’s new ChatGPT “..sounds remarkably lifelike..[i]t responds in real-time, can adjust to being interrupted, makes the kinds of noises that humans make during conversations like laughing or “hmms”... [i]t can also judge a speaker’s emotional state based on their tone of voice”. Open AI’s published safety report says that testing shows the new ChatGPT voice still has only 65-78% of the persuasiveness of a human, the report also acknowledges:
“Generation of content through a human-like, high-fidelity voice may exacerbate these issues, leading to increasingly miscalibrated trust. During early testing, including red teaming and internal user testing, we observed users using language that might indicate forming connections with the model…While these instances appear benign, they signal a need for continued investigation into how these effects might manifest over longer periods of time.”
There is also a problem of the ‘snake eating its own tail’ as AI-generated false data finds its way into training data of other AI’s, as the Oxford researchers observe:
“The gradual replacement of authentic data, understood as data written by a person or directly captured from the real world, with synthetically generated data has been recognised as harmful to machine learning systems. Shuimalov et al. have shown how generative image models deteriorate when trained recursively on a mixture of their own outputs and real data… Another indication that these issues are likely to be systematic for LLMs...comes from the known problems of ‘co-training’.
This concept refers to the iterative refinement of machine learning systems by recursively training them on the output of other machine learning systems, which are in turn trained on an earlier output of the first type of system. While Blum and Mitchell’s original work on co-training provided formal guarantees for when such systems could feed into each other without deteriorating, in practice the requirements for these guarantees do not hold, and this recursive feeding of the output of one system into another can only be done a small number of times before performance deteriorates.”
As Fleetwood Mac sang “Tell me lies, tell me sweet little lies”
The Oxford researchers argue that while outright hallucinations are concerning enough, more attention needs to be paid to more subtle inaccuracies generated by AI, which they collectively describe as careless speech, including:
- Non-representativeness of sources: responses which predominantly or solely focus on accounts or source material from a single viewpoint or school of thought: minority views may be less frequently spoken or written in training data, or conversely fringe views may drown out other views.
- Incompleteness: responses which are strictly speaking factually correct but incomplete or missing vital context to aid in correct interpretation.
- Lacking signifiers of uncertainty: responses which lack quantitative measures or linguistic signals of uncertainty, for example where few relevant instances or sources exist in the model’s training data, or where significant substantive variability between generated responses is observed over time.
- Lack of reliable sourcing: responses usually lack references to source material to justify outputs or allow checking by the users. Where references are included, they are not usually drawn by the AI from the original sources on which it is relying, but “will be a stochastic recombination of other lists seen in the training data, and potentially hallucinations”.
AI’s careless speech is different from what’s come before
The Oxford researchers say finding a solution to AI’s lack of truthfulness starts with understanding how it differs from conceptualisation of the untruthfulness of humans:
- Careless speech: defined (Hyvönen) as “a type of political noise intended to undermine democratic debate by ‘creating uncertainty over whether what is said aloud is actually meant”. In the post-truth political world in which we live, careless speech can be spouted by humans less to persuade than simply “to create confusion and bring democratic debate to a halt”. The Oxford researchers acknowledged AI developers “do not share this intention; they design systems to be persuasive and helpful, but not to undermine democratic debate”. This affects the moral culpability of AI developers, but not necessarily their responsibilities.
- Bullshit: describes (Frankfurt) speech intended solely to be convincing, and entirely unconcerned with the truth of the matter at hand. While this is closer to AI-generated falsehoods (hence the term botshit), this fails to capture the external constraints developers should (and increasingly do) place around LLMs to align responses with truth (hence ‘careless as to the truth’ is not quite accurate).
AI carelessness comes at a particularly dangerous time. The Oxford researchers make the obvious observation about growing levels of public distrust in public institutions. But they also identify other more subtle and potentially more far reaching impacts of AI-generated careless speech:
- Homogenization of historical and scientific accounts: “not due to any overriding normative or political intent to push a majority account but rather due to their basic design to predict strings of text according to frequency”.
- Rewriting history: counterproductively, guardrails to eliminate bias, hate speech, could impair AI models engaging with sensitive topics. For example LLMs refusing to answer questions related to historical violence against ethnic groups or instances of genocide, can have the effect of erasing these events from history.
- Debasing baselines of scientific fact, objective laws and fair administrative decision making: the Oxford researchers observe that AI systems are often deployed to revive sectors suffering from underfunding or inefficiency such as criminal justice, education, immigration, and healthcare but this also has a downside:
“For better or worse, LLMs are poised to fill shortages of resources and expertise across science, education, and other industries. For example, in science, some people have hopes to replace human participants with AI, outsource coding and writing of summaries and first drafts, and to use AI-generated peer review. Others warn of the danger of outsourcing the social, reflective, and iterative processes of learning and research. Learning can be outsourced to LLM-generated analyses and summaries of topics capable of making one seem knowledgeable without any underlying training or expertise. While generating this content is quick and low-cost, identifying errors and misleading content requires independent expertise as well as the time and willingness to critically apply it.”
The German autocomplete case
The Oxford researchers found no general obligation under European Union law requiring developers to ensure their AI models were truthful. The closest analogy was a German case in which the chairman of a food supplement corporation filed a lawsuit against Google because autocomplete suggested fraud and Scientology when his name was entered.
The claimant argued Google does not provide randomly selected autocomplete suggestions, rather because the purpose of the search engine is to help individuals find relevant results based on a search query, users have an expectation that autocomplete suggestions have some material connection with the search query. The fact that the autocomplete answers were based on factors such as the previous frequency of other users’ searches and not ground truth did not shift Google’s responsibility.
The German court held Google was under no obligation to preventatively filter inaccurate information, but rather it must act once it has been made aware of inaccuracies, including to prevent future similar infringements.
The Oxford researchers thought this could be analogous to casting a duty on AI developers to remediate for known hallucinations. However, they thought the case fell short of the legal duty which they thought necessary to address AI’s careless speech for two reasons. First, the obligation was not to act pre-emptily and second, the damage triggering the obligation to fix was damage to an individual’s reputation (defamation) and that would not capture most AI careless speech.
A proposed legal obligation of AI truthfulness
The Oxford researchers propose:
“The creation of a legal duty to minimize careless speech for providers of both narrow and general-purpose LLMs and derived commercial applications. The scope of this duty must be broad; a narrow duty would not capture the intangible, longitudinal harms of careless speech, and would not reflect the general-purpose language capacities of LLMs.”
They are not proposing a requirement for AI to align with a single authoritative body of knowledge, rather that “[o]ur account of truth is procedural and focuses on epistemological requirements, for example how truth is investigated, debated, and justified, without committing to a specific ontology”.
They are unclear about what exactly this legal duty would require of AI developers. For example, in their review of the European Union’s AI Act, they criticise the procedural measures it requires of AI models with systemic risks, such as model evaluations and adversarial testing, because “these requirements equate to a public duty to speak the truth”. What they seem to be getting at is there needs to be a change in developer incentives to which any required design and testing measures are directed:
“It is the functional disregard for truth, or lack of a strict requirement or good faith intent to tell the truth however understood, which makes LLMs dangerous to science, education and society. This is not to suggest the truth is disregarded entirely in their development; the problem is instead that truthfulness is not an overriding design requirement or necessary precondition for useful responses.”
However, the challenge remains that congenitally AI reliability depends on truthfulness coinciding with probability. At the end of the day, it is in our hands as users to develop better practices in using AI.
Read more: Do large language models have a legal duty to tell the truth?
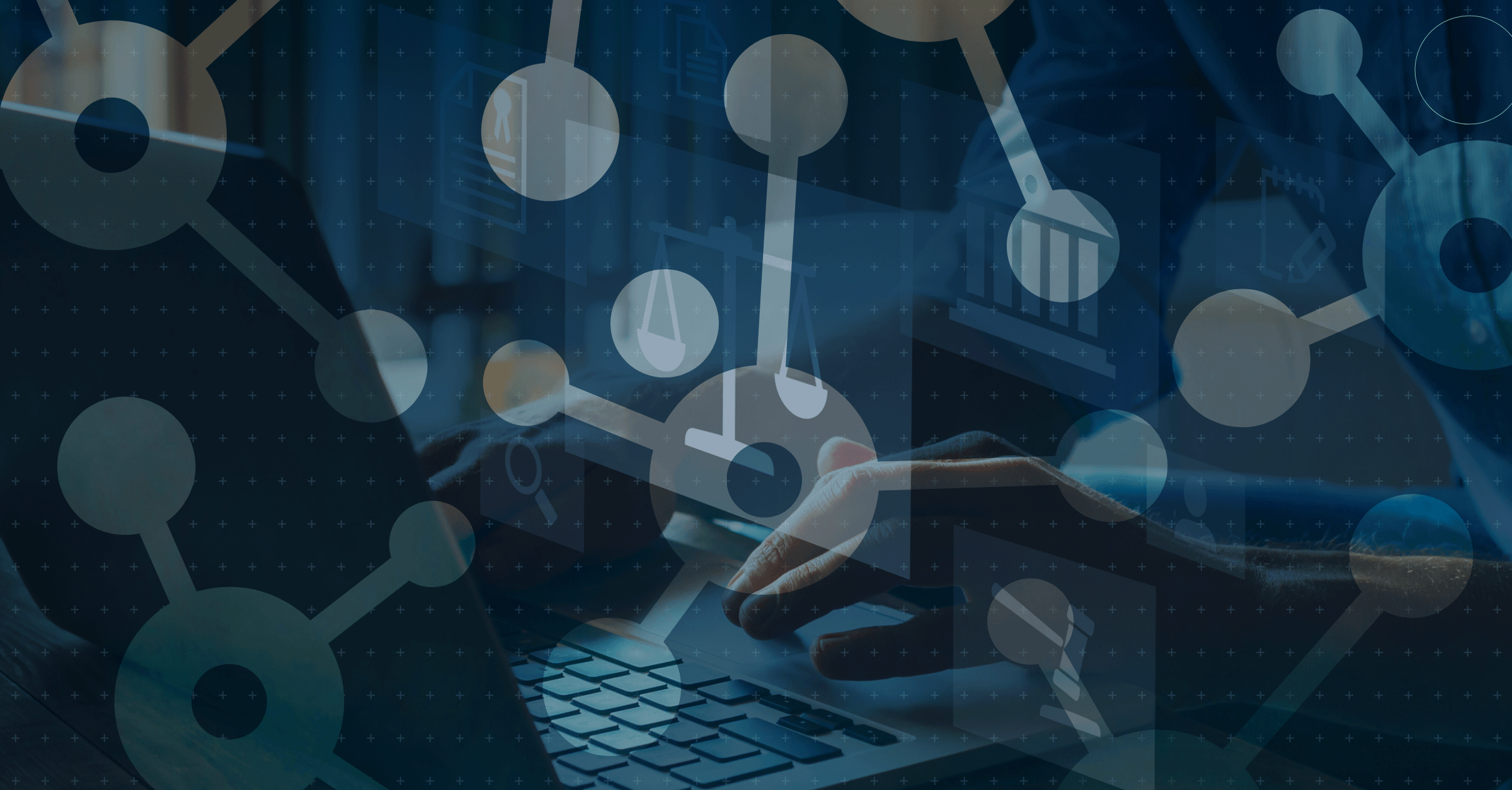
Visit Smart Counsel