In early March 2024, the UK Government released a report by an independent expert panel into equity in medical devices.
The inquiry was triggered by concerns that a medical device called a pulse oximeter, which clips on the end of your finger and estimates the level of oxygen in the blood by sending light through the skin, tends to overestimate oxygen levels in non-White patients. During the COVID pandemic, this could have delayed hospitalisation and use of oxygen for non-White patients.
While the pulse oximeter is old, ‘dumb’ medical tech, the report took the opportunity to consider broader issues of equity in the design, training, and clinical use of medical AI:
“AI-enabled medical devices are entering the market at an unprecedented pace. Almost under the radar, their acceptance as ‘routine’ could obscure their potential to generate or exacerbate ethnic and socio-economic inequities in health…. When AI is built around a ‘standard patient’, other patients can be harmed. The assumptions built into technology around a standard patient – typically White, male, relatively affluent and born in the UK.”
Defining bias
While the report addresses the specific issue of racial and ethnic bias "as these are more obviously likely to arise in the medical devices", it concluded that "ultimately the biggest driver of health inequity in the UK by far is socioeconomic disadvantage (regardless of ethnic group)".
As its touchstone, the report said that equity required that medical devices:
- be available to everyone in proportion to need;
- support the selection of patients for treatment based on need and risk; and
- function to the same high standard and quality for all relevant population groups. If there are unavoidable differences in performance among some groups, these need to be understood and mitigated, such as in how the device is calibrated
The report found clear current ‘violations’ of these principles of equity in the NHS:
“…but from what we could discern it was largely unintentional (for example related to the physical properties of optical devices on darker skin tones or unrepresentative datasets in AI algorithms), compounded by testing and evaluation in predominantly White populations. Some of the biases we found were even well-intentioned but misguided, such as the application of race correction factors based on erroneous assumptions of racial or ethnic differences...”
The report advocates a ‘distributive justice’ (i.e. equality of outcomes) approach, which contrasts with the ‘equality’ approach often taken in AI model development:
“...AI developers mistakenly equate equity with equality, and come up with adjustments to algorithms that simply equalise performance or outcomes between socio-demographic groups, taking no account of differences in healthcare need. These attempts at equalisation can even result in ‘levelling down’, where fairness is achieved by making every group worse off. This violates the central tenet of health equity, which is to level up – reduce the health gap by bringing the health of worse off groups closer to those who are better off.”
How does bias creep into the health system?
The report identified four ‘entry points’ for bias in the medical device ecosystem, as depicted below:
The report observes an ‘unvirtuous circle’ in medical technology:
“…in which injustice and health inequities originating in the real world are carried into the production and use of medical devices, where further bias may be introduced at various points in the device lifecycle. It can come full circle when using specific medical devices may, in extreme circumstances, bring about injustices in wider society.”
Starting in the real world, long-established socioeconomic and ethnic inequities generate:
- higher levels of ill health
- the 'inverse care law’ – historic under-provision in disadvantaged localities and for less privileged socio-demographic groups
- poverty and discrimination making access to services more difficult
- poorer living conditions and nutrition making it more difficult to maintain a good quality of life during chronic illness and to recover from periods of ill health
The most obvious risk is that these patterns, prejudices, and attitudes can be encoded in datasets on which medical AI is trained. But there are, in the report’s view, much wider consequences for the medical device ecosystem, in particular, in ‘problem selection’ – how health problems are selected and prioritised for AI-related development.
Moving onto the problem of ‘baked-in’ discrimination in data, this skewing of data from the real world can be compounded by medical research because "[r]esearchers may overlook some population groups during data collection, which tends to favour the interests of privileged and dominant groups, leading to sampling and selection bias." Worse still, attempts by well-meaning researchers to fill in the gaps with statistical correction methodologies themselves can embed stereotypes: for example, a test for elevated levels of creatinine in the blood used to diagnose chronic kidney disease until recently incorrectly needed a higher level for diagnosis for Black patients than for White, resulting in a withholding of treatment for Black patients until their kidney disease is more advanced.
Moving then to product design, this problem has been around for some time and the report gives a litany of examples:
- a ventilator enhances the delivery of oxygen to the lungs. Although women’s lungs tend to be smaller, the default settings on the ventilators are for men. The clinical team needs to remember to manually adjust the settings to prevent the machine from damaging a female patient’s lungs.
- Glaucoma, which can cause loss of vision and blindness, is diagnosed using medical devices that test eye pressure, retinal thickness, and visual field. While the retinal nerve fibre layer is, on average, thicker in people of Asian than European ancestry, scanners typically have only one reference database, from a European ancestry population. This can result in the device missing at an earlier point that the readings for a non-White person are outside the normal range relevant to them.
- use of the pre-AI dermoscopes requires training of clinicians to recognise skin disorders and lesions in darker-skinned people because it is recognised that the clinical signs may differ according to skin tone. However, when it comes to training AI-based dermoscopes, recent studies have identified a lack of photographs in textbooks of skin disease and skin lesions affecting darker-skinned individuals and a dearth of darker-skinned populations in clinical and research studies.
- another optical medical device is a transcutaneous bilirubinometer used to test newborn babies for jaundice caused by elevated serum bilirubin. The device estimates the concentration of bilirubin in the baby’s blood by using optical spectroscopy to measure the amount of light absorbed by the bilirubin. Studies suggest bilirubinometers tend to overestimate the concentration of bilirubin in non-White babies compared to White babies, potentially triggering an unnecessary pathway of more invasive tests and treatment for non-White babies.
Finally, at the last stage of the unvirtuous circle when medical devices are put in the hands of clinicians, further biases may arise.
“Much of today’s education, training and professional development does not equip them with the knowledge and skills to identify and address equity issues, while clinical guidelines may not specify mitigating actions.”
The report contrasts the lack of research, monitoring and regulation of AI medical devices once released into a clinical environment with the high level of post-deployment surveillance of new drugs.
What’s on the horizon?
The report expressed concern that the medical profession and regulation are not up to the task of keeping pace with AI:
“…the exponential increase in AI-driven applications in medical devices has far surpassed any increase in regulation of AI used to support clinical decision making and AI-derived predictive analytics, including in genomics. There is a real danger that innovations in medical device technology, whether in optical devices, AI or genomics, will not only outstrip the growth in our health professionals’ AI literacy and skills but will also exacerbate inequity, with potential to change the foundations of the doctor–patient relationship in unpredictable ways.”
As an example of the challenge of rapid technological change, the report identified concerns around applications and devices in genomics utilising polygenic risk scores (PRS) tests. PRS is a genome-related test that aggregates information across multiple disease-associated single nucleotide polymorphisms or SNPs (often millions) into a single combined score – a PRS –to assess an individual’s genetic predisposition to a disease or trait.
Already many internet businesses providing ancestry testing have branched into offering consumers, for a small extra fee, ‘medical testing’ through secondary analysis.
The report expressed the following concerns with the on-coming train of PRS:
- the major genetic datasets employed by PRS are drawn from populations that are overwhelmingly of European ancestry. In the UK Biobank, for example, which is the largest and most widely used dataset of its kind in the world, 94.6% of participants are classed as White and only 1.6% are classed as Black and 1.6% South Asian.
- For many common diseases, non-genetic factors such as smoking, poor nutrition, socioeconomic deprivation and inadequate living and working conditions, matter more than a person’s genetics. An over-emphasis on PRS results may cause unnecessary anxiety amongst consumers, divert attention away from laudable public health programs, and blunt efforts to address the too-often-neglected social inequity and injustice in health care systems.
- while PRS has not (yet) been formally introduced into the NHS, an immediate policy response is required because "they are already being used haphazardly with little or no regulation in other countries, and they are trickling into the UK through commercial, direct-to-consumer routes without any regulation or support for the people who receive this sort of information".
Recommendations
The report’s recommendations on how to better achieve equity in medical devices echo those made in the recently released US Government’s guidelines on addressing bias in medical AI, but with a sharper, more detailed focus:
- on the specific problem of optical oximeters, as a workaround for current devices in circulation, clinicians and patients at home should be trained to pay more attention to the variation in readings across multiple applications and not make decisions on absolute thresholds. For new optical devices coming to market, standards for approval should include sufficient clinical data to demonstrate accuracy overall and in groups with darker skin tones (but the report conceded that the technological solution to this issues has a way to go).
- More generally on medical AI, developers, research funders, regulators and users of AI devices must openly recognise and address the limitations of many commonly used datasets by obtaining more diverse and complete data. This will include making a concerted effort to build trust with minority and disadvantaged groups.
- regulators should:
- require developers and manufacturers to report the diversity of data used to train algorithms;
- provide guidance that helps manufacturers enhance the curation and labelling of datasets by assessing bias, being transparent about limitations of the data, the device and the device evaluation, and how to mitigate or avoid performance biases; and
- provide guidance that helps manufacturers enhance the curation and labelling of datasets for bias.
- require developers and manufacturers to report the diversity of data used to train algorithms;
- the UK Government and the medical profession must:
- ensure professional training includes the potential for AI to undermine health equity, and how to identify and mitigate or remove unfair biases;
- produce material for AI developers about equity and systemic and social determinants of racism and discrimination in health; and
- ensure that clinical guideline bodies identify how health professionals can collaborate with other stakeholders to identify and mitigate unfair biases that may arise in the development and deployment of AI-assisted devices.
- ensure professional training includes the potential for AI to undermine health equity, and how to identify and mitigate or remove unfair biases;
- the medical device approval process in the UK should treat all medical AI, "other than the simplest and lowest risk technologies", as falling into the medium risk or higher categories.
- specifically on PRS:
- the UK Government should fund a broad programme of research and consultation with the public, patients, and health professionals to fill the gaps in knowledge and understanding concerning PRS.
- professional bodies and health education bodies should develop guidance for healthcare professionals on the equity and ethical challenges and limitations of applying PRS testing in patient care and population health programmes.
- the UK Government should fund a broad programme of research and consultation with the public, patients, and health professionals to fill the gaps in knowledge and understanding concerning PRS.
Read more: Equity in medical devices: independent review - final report
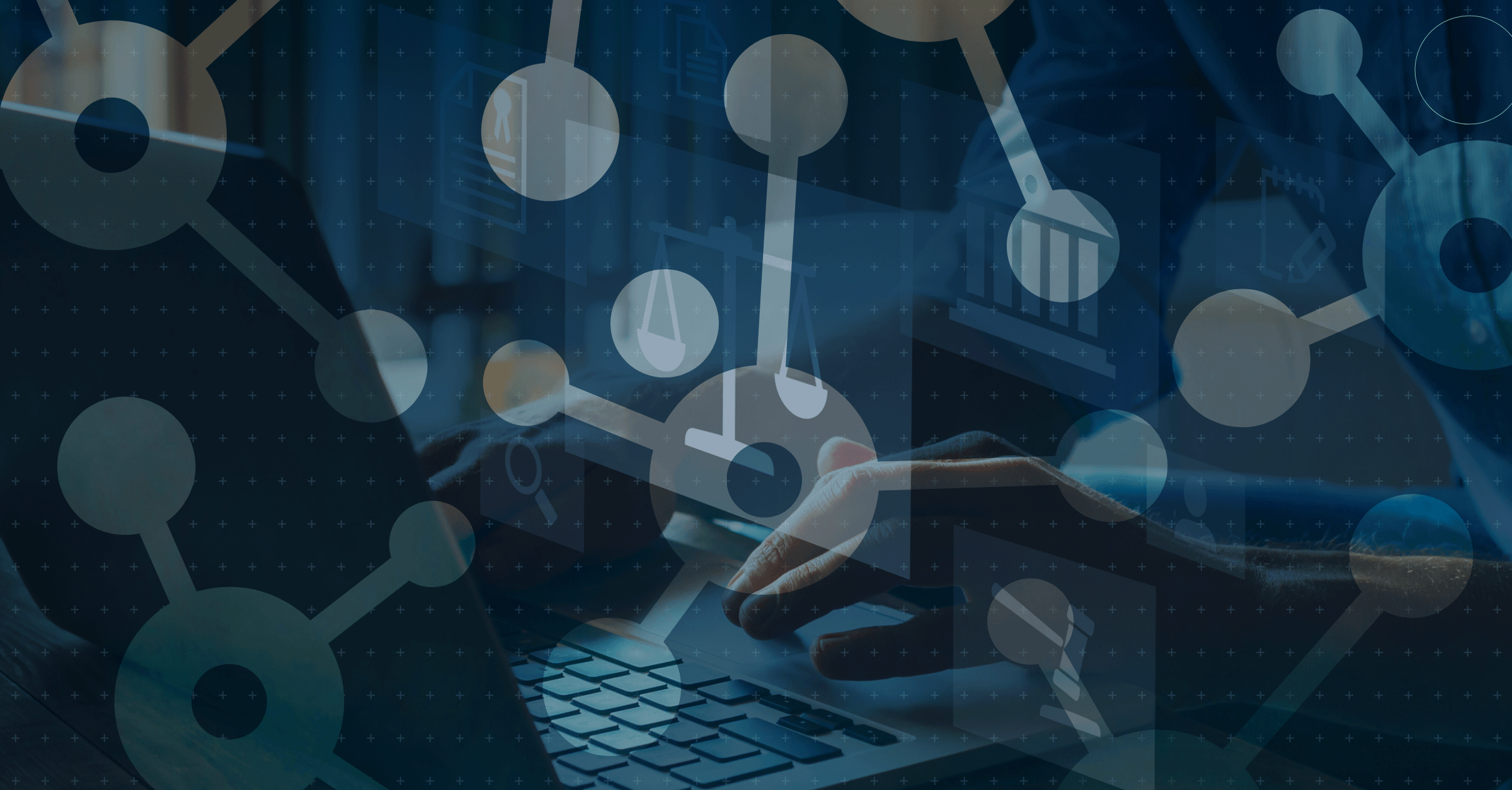
Visit Smart Counsel