How do you pick your next song? New albums from your favourite artists? Hot hits playlists? Maybe just seeing what your friends are listening to? Or perhaps you like to hand over the reins to your music streaming service of choice (streamers) and let it choose your next song, whether it be smart suggestions based on what you were just listening to, or a playlist curated just for you. If you’ve ever fit into that last category, your music listening, as you may already appreciate, has been shaped by Artificial Intelligence (AI).
Depending on your perspective, AI-powered music recommendations mean our music tastes are either being unwittingly shaped by unseen AI forces in favour of certain interests, or, alternatively, doors are being opened to new worlds (artists, songs) that would be otherwise near undiscoverable by traditional means.
A recent UK Government report has considered the impact of recommendation algorithms, grappled with themes such as the potential for algorithmic bias, and canvassed the perspectives of music artists (by polling 102 artists) and listeners (by polling ~4000 UK adults).
Recommendation Systems?
To start, it’s useful to understand how these recommendation systems work, using one of three approaches:
Collaborative filtering - which works by recommending songs that other people listening to the same songs/artists as you like (e.g., by “liking” a track);
Content-based approaches - which work by recommending songs/artists based on feedback provided by experts or audio analysis and the actual content of the music (e.g., artist and song title); and
Contextual approaches - which work by recommending songs based on contextual factors (e.g., the device you listen on, day of the week it is, the weather at the time of listening etc.) to make recommendations.
Collaborative filtering - which works by recommending songs that other people listening to the same songs/artists as you like (e.g., by “liking” a track);
Content-based approaches - which work by recommending songs/artists based on feedback provided by experts or audio analysis and the actual content of the music (e.g., artist and song title); and
Contextual approaches - which work by recommending songs based on contextual factors (e.g., the device you listen on, day of the week it is, the weather at the time of listening etc.) to make recommendations.
But how recommendation systems specifically work in practice remains an open question, with streamers reluctant to say too much, for fear of losing one of their main competitive edges, and also to prevent artists from 'gaming' the systems (if you can't understand the system, then you can't exploit it - at least, that's the thinking).
What we all fear is happening
The natural consequence of that lack of transparency is mistrust. And if there's one key takeaway from the Report, it's that we, the people, aren't a particularly trusting lot; ~89% of artists and ~49% of listeners polled considered that AI-powered recommendations could result in bias and unfairly prioritise certain music.
Creators were particularly concerned that AI was reshaping music around them: 85.3% of creators expressed concern that algorithmic bias could lead to music from certain genres being prioritised over others, while 67.7% of respondents are concerned that recommendation algorithms could lead to certain demographic groups (e.g., different ethnic groups, genders etc.) being prioritised over others.
Creators also were concerned that major labels are 'gaming' the algorithms and biasing them towards artists they represent, or that streamers might promote music they hold rights over to reduce remuneration they have to payout.
As for consumers, fewer than one in five (18%) said they completely understand how these recommendations work, with one quarter (25%) reporting that they do not understand how they work at all. Two thirds of respondents who currently use a music streaming service agree that they would like more information about what types of data music streaming platforms collect. Among those who had a streaming service in the past, a significant minority (42%) reported that a lack of information about what data music streaming services collect was a factor in their decision to stop using them.
But is AI playing to a different tune?
Though, while public cynicism regarding these recommendation algorithms is no short supply, the Report concluded that, while the data is limited, it was unable to prove or disprove such bias.
If anything, recommendation algorithms may have positively contributed to the observed shift towards streaming less popular songs and artists in the UK since 2018. An earlier study by the UK IP Office drew on comprehensive UK streaming data from a sample month (October) for each of the years from 2014 to 2020. While the study found that the rate of concentration of big name artists had been consistently high for some time, more recently there has been a noticeably longer and more significant tail in new music and new artists: for example, the percentage of total streams accounted for by the top 0.1% of tracks increased from 40% in 2014 up to 46% in 2016, but then declined quite steeply back to 41% in 2020.
While the Report did not speculate as to why, we think this can be put down to two factors. First, there is a vast, rapidly expanding ocean of music out there on which recommendation systems can feed, much of it DIY or independently published. According to Luminate , a leading entertainment data and insights company, an average of 98,500 separate music files were distributed daily to audio and/or video streaming services between September 1 - October 18, 2022, yet just 4% of those were distributed by the three major global music companies, Universal, Sony and Warner. To avoid being swamped, the record companies are ”cast[ing] our nets deeper and deeper” to bring increased volumes of independent music into their distribution system .
But the volume of new music is not the full answer because in 2022 67.1 million audio tracks on music streaming services (representing over 42% of the entire catalogue) attracted 10 or fewer streams apiece, and 38 million were played zero times . So, our second factor is the way recommendation systems can work. Take Spotify as an example. When a new artist uploads their first song to the service, it would not show up in the recommended lists if the Spotify AI relied entirely on natural language processing (NLP) of the Internet to identify music trends or on collaborative filtering from Spotify listeners, since in both cases there is little or no data about the song. But Spotify has a third algorithm that analyses each uploaded song to create a spectrogram of the song — its volume, timbre, size, harmony, etc. Then, it compares them with the characteristics of other songs and finds similar ones, after which the track goes to the playlist "Discover Weekly".
Yesterday all our troubles weren't so far away
One of the most interesting perspectives from the Report is that attempts to shape our listening habits and music tastes is not new. Digitally-based recommendation systems have become a new type of, to take the term from the Report, 'cultural intermediary' affecting the music listeners hear, sitting side by side with traditional 'cultural intermediaries' such as radio presenters or music critics.
The Report makes the good point that criticism of digitally-based recommendation systems overlooks the tight grip which ‘traditional recommendations systems’ had on our music tastes. When we consider how listeners would discover music prior to the advent of digital streaming services, there can be no doubt that there was an invariable bias towards chart-listing music (the ‘Top Ten’ charts); radio, TV, magazines and newspapers would report on and feature only the top songs, clearly advantaging artists with significant financial resources or came to the attention of the radio or TV hosts (Molly Meldrum’s ‘discovery’ of ABBA).
Similarly, as the Report notes, the music industry has historically privileged certain demographics - in the UK one only has to look at the hit artists of yesteryear to see the bias towards "white males" the Report calls out (e.g., The Who, The Rolling Stones, The Beatles, Queen and The Cure all fit neatly into this categorisation). To extent that recommendation systems seem to portray a demographic bias, it may be unfair to blame the algorithms if they are merely reflecting the historical bias entrenched within the industry.
Challenger radio stations such as the Australian Broadcasting Commission’s 2JJ were established to break the grip of the human ‘recommendation systems’ on broadcast music (the first song played on 2JJ was Skyhook’s You Just Like Me ‘Cos I’m Good in Bed, which had been uniformly banned by commercial radio).
So where does that leave us?
It seems that recommendation systems, are, at the very least, no worse in terms of 'unfair' bias than other, more traditional forms of discovering new music. But at best, given the vast ocean of music that they can scan and process compared to the human intermediaries of the past, they could live up to the promise of opening the door to new artists and music that otherwise would have remained inaccessible.
Data scientists for streamers noted they are actively trying to counter any popularity bias. The Report commented that this makes sense from a purely commercial standpoint; in an age where streaming services increasingly gain parity with each other in pricing, music catalogue, and unique features, the strength of their recommendation systems stands out as a core differentiator. It's in streamers' interests for their recommendation systems not to regurgitate the same songs, but to offer wholly novel recommendations to their listeners, to improve user satisfaction.
Recommendation systems also don't hold the sway over listeners that you might think - according to streamers, only ~30% percent of user listens are ‘guided’ by recommendation systems whereas ~70% of listens continue to be user led. Sure, there are some generational differences: 36% of 18-25 year olds are ‘guided’ by streaming service recommendations, compared to 9% of over 65 year olds. Even so, the key the takeaway is still that the majority of listens are influenced by other, more traditional factors, such as radio, family and friends and social media when it comes to new music recommendations.
That hasn't stopped artists from trying to take advantage of recommendation systems, whether it be in the way they release albums or even create songs. A majority of surveyed creators (53.5%) candidly acknowledged that they, or their representatives, have changed the way they release music, in order to try and increase the likelihood of it being recommended. However, a much smaller percentage (16.7%) reported that they have changed the way that they make music, in order to increase the likelihood of recommendation across streamers. The Report gives the example of artists trying to create an attention-grabbing first 30 seconds, given that streamers tend to only remunerate artists for listens >30 seconds.
But it would be unfair to give recommendation systems too much credit in affecting the evolution of music. There have always been new factors affecting music's evolution; today it may be artists chasing TikTok fame by focussing on short, catchy and easily loop-able refrains, and in the 2000s we saw the same thing during the 'ringtone era'. Instead, it may make more sense to place recommendation systems as a factor, yes, but one of many, that influence the evolution of music.
Final Thoughts
It’s clear that AI isn’t going anywhere and recommendation systems powered by AI are here to stay. The Report recommends that more transparency in how streaming services communicate about recommendation systems could help ease feelings of discontent, and better foster trust between parties in the music ecosystem - even an industry-based standard model.
So, next time your ears perk up as your chosen streamer automatically starts playing a song you didn't queue, just know that AI has impacted your day in at least a very small way. Whether that impact will be larger tomorrow, well, we'll just have to wait and see. Whatever the case, one thing’s for certain - best keep your ears tuned.
Read more: The impact of recommendation algorithms on the UK's music industry
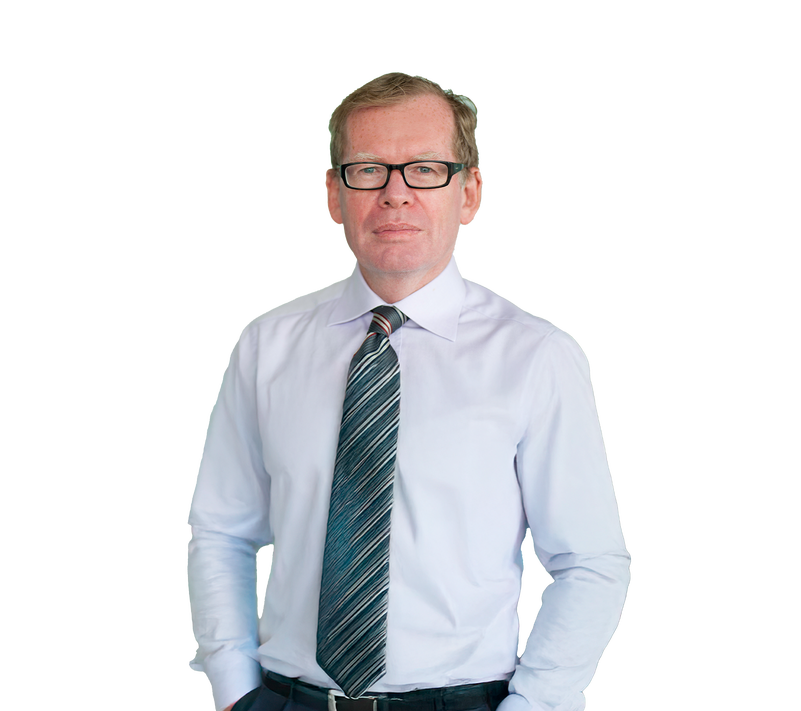
Peter Waters
Consultant