In her Harvard Law and Technology Journal article, Alice Xiang, Sony’s Global Head of AI Ethics, argues that, in the headlong rush to regulate FRT, there is “[a]n under-appreciated tension between privacy protections and bias mitigation efforts”.
Xiang applies her analysis broadly to ‘human-centric computer vision (HCCV)’ which she defines as AI systems that rely on images of humans for their training and test data. FRT is a subset of HCCV.
The problem
Studies have attributed the biases in HCCV AI to a lack of diversity in the datasets used to train these commercial AI systems. Reducing bias in HCCV systems requires collecting a large, diverse, and candid image datasets, which can run counter to privacy protections.
But as Xiang points out, bias in HCCV systems is more nuanced than not seeing enough faces. Algorithmic bias boils down to two problems: (1) lack of representation; and (2) spurious correlations.
On the first problem, humans have trouble recognising faces of races other than their own if they have not had enough exposure to those other races: called the “other race problem” or less politely, “they all look the same to me”. AI has much the same problem: algorithms developed in Western countries perform better for Caucasian faces while conversely algorithms developed in East Asian countries perform better for East Asian faces.
On the second problem, AI can make spurious correlations because it ‘divines’ misleading patterns from the training data, often due to societal biases in the training data. For example, AI is more likely to incorrectly predict that an individual in a photo is female if the background is indoors and the reverse for outdoor images, perpetuating long-standing stereotypes of women inhabiting domestic spheres and men inhabiting public spheres.
Past solutions have run aground on privacy concerns
There has been a troubled history of AI developers trying to address bias problems by ‘harvesting’ faces of real people to expand and balance out AI’s training data.
Xiang gives the example of IBM’s “Diversity in Faces” (“DiF”) dataset which was initially received positively for being far more diverse and balanced than previous face image datasets. DiF, however, soon became embroiled in controversy once journalists highlighted the fact that the dataset consisted of images ‘scrapped’ from from Flickr. While the Flickr images had Creative Commons licenses covering the copyright of the images (as part of the Flickr terms of use), a US class action argued that the plaintiffs had not consented to having their images used in facial recognition training datasets.
The privacy implications of using real human faces for training data goes deeper than just the use of an individual facial image but requires sensitive information about personal attributes, such as the individual’s racial or gender identification, to allow AI to do the matching. As Xiang says “to even discern whether a training dataset is diverse, we need a taxonomy of demographic categories, some notion of an ideal distribution across that taxonomy, and labels of these demographic categories.”
It can be an even rockier path when the AI designers, if they do not have the variable for race or ethnicity, use computational methods to derive labels for different facial features to indirectly capture differences across race, including metrics for skin colour and craniofacial areas. Xiang points out that “these approaches do not reflect the sociological nature of demographic labels and could be misused, as we have seen in the pseudoscience of physiognomy, which focuses on quantifying physical differences across races.”
Different legal treatment of ‘unseen’ vs ‘mis-seen’
Part of the tension between privacy rights and bias mitigation in AI lies in the different legal rationales which underpin each.
Xiang argues that ‘[w]hile being “seen” by an HCCV system without informed consent is considered, under some privacy laws, to be a harm in and of itself, being “mis-seen” is only considered to be harmful if it leads to a separate legally cognizable harm, which will usually be an action for discrimination in defined areas, such as housing, employment or financial services.
While limiting the scope of anti-discrimination remedies to specific domains may be appropriate for conduct by human actors, she says we need to relook at algorithmic discrimination because the growing proliferation of HCCV in everyday life can mean that even small or subtle biases might accumulate into substantial harm. She gives the following vivid example of how hard a day can be for a person ‘mis-seen’ by AI:
"Upon waking up, you check your phone, but it does not recognize you, so you have to manually input your passcode. Taking public transit to work, you try to use the facial recognition system to pay your fare, but it does not recognize you, so you must go through a special line with a human verifier and arrive late to work. You join your colleagues for coffee at a cafe, but again the payment system fails to recognize you. You are embarrassed as the automated system says your face does not match the bank account you are trying to access, and you have to ask the cafe staff to give you another method of payment. They, unfortunately, do not have any other methods of payment, so you need to ask a colleague to cover your tab. When you and your colleagues return to the office, you are unable to enter the building because the security system does not recognize you as one of the employees. While your colleagues are waiting for you, you call for a security guard to help you enter the building. The security guard is suspicious of your claim that you work in the office — the picture in the employee data-base looks like it could be someone else, and the AI system works extremely well for everyone else. Fortunately, your colleagues vouch for you, and the security guard lets you in. At the end of the workday, you stay late, after your colleagues have left, to finish a project. The lights and AC turn off, as the AI-enabled AC and lighting systems do not detect any people in the office. Sitting in the darkness, you are confronted with your own invisibility."
Turning to privacy laws, Xiang says that, while we may endorse privacy as a fundamental right, privacy law is “notorious for the ambiguity around the specific harms it envisions”. One of the main concerns identified in the US court cases and regulatory decisions about use of real faces and other biometric data is the risk of mass surveillance. However, she argues this surveillance concern is over-generalised for HCCV for two reasons:
- the harm of mass surveillance is tied specifically with the breadth of deployment of HCCV rather than the breadth of the data used to develop it. The fact that AI has been trained on a large data set including your face does not mean that, when deployed, the AI can or will be ‘looking for you in the crowd’. Xiang does acknowledge that the line between training and deployment can blur, such as when the HCCV is being retained after deployment; and
- not all forms of HCCV facilitate mass surveillance. Face, body, and object detection or classification do not directly enable mass surveillance since they do not involve identifying individuals: e.g. if FRT is used only locally on your phone to sort your photos, and the matches are not shared with the company or any-one else.
The stricter rationale for privacy is the right to be ‘left alone’, which does not require a harm to be established. Applying this rationale to HCCV, a US court has said that privacy law protects an individual’s “control of information concerning his or her person,” such that lack of control over one’s biometric information itself constitutes the harm.
Xiang notes that if this is to be the basis of how privacy law is applied to HCCV training, then there will be an “irreducible paradox” in efforts to design less biased HCCV between the simultaneous desire to be “unseen” yet not “mis-seen”.
What are her solutions?
Xiang lists out some possible approaches to balancing privacy and bias mitigation in HCCV:
- the most obvious would be to comply with privacy law and obtain consent from people for use of their facial images in training HCCV. However, Xiang notes the practical challenges, given the need for millions of faces in a HCCV data set and that in order to ensure diversity, the data set would need to be collected across many different jurisdictions with very different privacy rules. While the global social media companies have the reach to collect the data, the Flickr case shows the challenges in meeting the new, more specific requirements of informed consent in many jurisdictions;
- pay ‘crowd workers’ to upload images of themselves based on particular specifications (e.g., one front-facing photo, one side-facing photo, one photo indoors, one photo outdoors). The problem is that these ‘selfies’ look staged. Computer vision works better when trained on “in the wild” images that appear to be taken in a wide variety of everyday scenarios — similar to the contexts a deployed HCCV system would be working within;
- use publicly available databases, including photos of celebrities (the sheer number of photos of an indiviudal celebrity in different settings can help in training). However, in addition to the barriers to non-whites participating in entertainment, politics etc, uncovering bias also can be difficult since existing publicly available datasets typically do not include people’s self-reported demographics, so researchers or developers who want to ensure dataset diversity then have to guess or estimate the demographics;
- Some scholars in the ‘algorithmic fairness community’ are pushing for an approach of participatory design — methods that engage stakeholders who use or are affected by technology in its design to build greater trust between the data subjects and the data collectors. However, Xiang says the key difference between building family archives, where this participatory model has been applied, and datasets for AI is the lack of incentive for most people to contribute to AI datasets: “[w]hile contributing to an archive can be seen as an honour, a way to preserve the history of your family or community, contributing to an AI dataset is viewed with wariness”. Hence the Flickr case;
- Shift the responsibility for data collection and storage from private companies to third-party actors (governmental or non-governmental) that might be more trusted for data collection. However, Xiang acknowledges that, even if wariness of government can be overcome, this solution only directly tackles the problem of trust, it does not necessarily solve the other challenges, such as how to get consent on a mass scale;
- Waiting for the (inevitable) technology solution – which may not be long given how fast things are moving in AI. Synthetic image generation can be used to generate images of people who are not real by modifying specific features of an individual (e.g., skin tone, hair length, or perceived gender): in effect, AI “hallucinating” new people. But as Xiang notes, the problem remains that “images of real people are typically used somewhere in the pipeline of creating images of synthetic individuals”, and hence the bias risks remain.
Xiang canvassed two legal changes which are, in a sense, two sides of the same coin:
- on the AI developer side of the coin, carving out from privacy laws a narrow exception that allows use of biometric data without consent to verify the lack of bias in an AI training data set, such as the proposed EU AI Act does.
- On the individual’s side of the coin, increase the protections against being “mis-seen”: i.e move away from the need to show harm flowing from being ‘mis-seen’ and make being ‘mis-seen’ itself a harm, levelling up with the privacy-based right not to be ‘seen’. Xiang says that this could be a general right for HCCV systems to have a minimum performance level (i.e. based in the law of negligence or product liability) or a new an anti-discrimination right for systems to not have a significantly disproportionate performance for one’s subgroup. This new right would provide more incentive for AI developers to directly address issues of algorithmic bias. Xiang acknowledges that “this would not directly solve the informed consent challenge posed by privacy laws, but creating such a right would better balance the ethical trade-offs around data collection.”
Xiang points out that there is no avoiding finding a balance between ‘unseen’ vs ‘mis-seen’ . While some jurisdictions have banned more controversial uses of HCCV, such as in policing, HCCV technology is rapidly spreading through our daily lives.
Read more: Being “seen” versus “mis-seen”: tensions between privacy and fairness in computer vision
KNOWLEDGE ARTICLES YOU MAY BE INTERESTED IN:
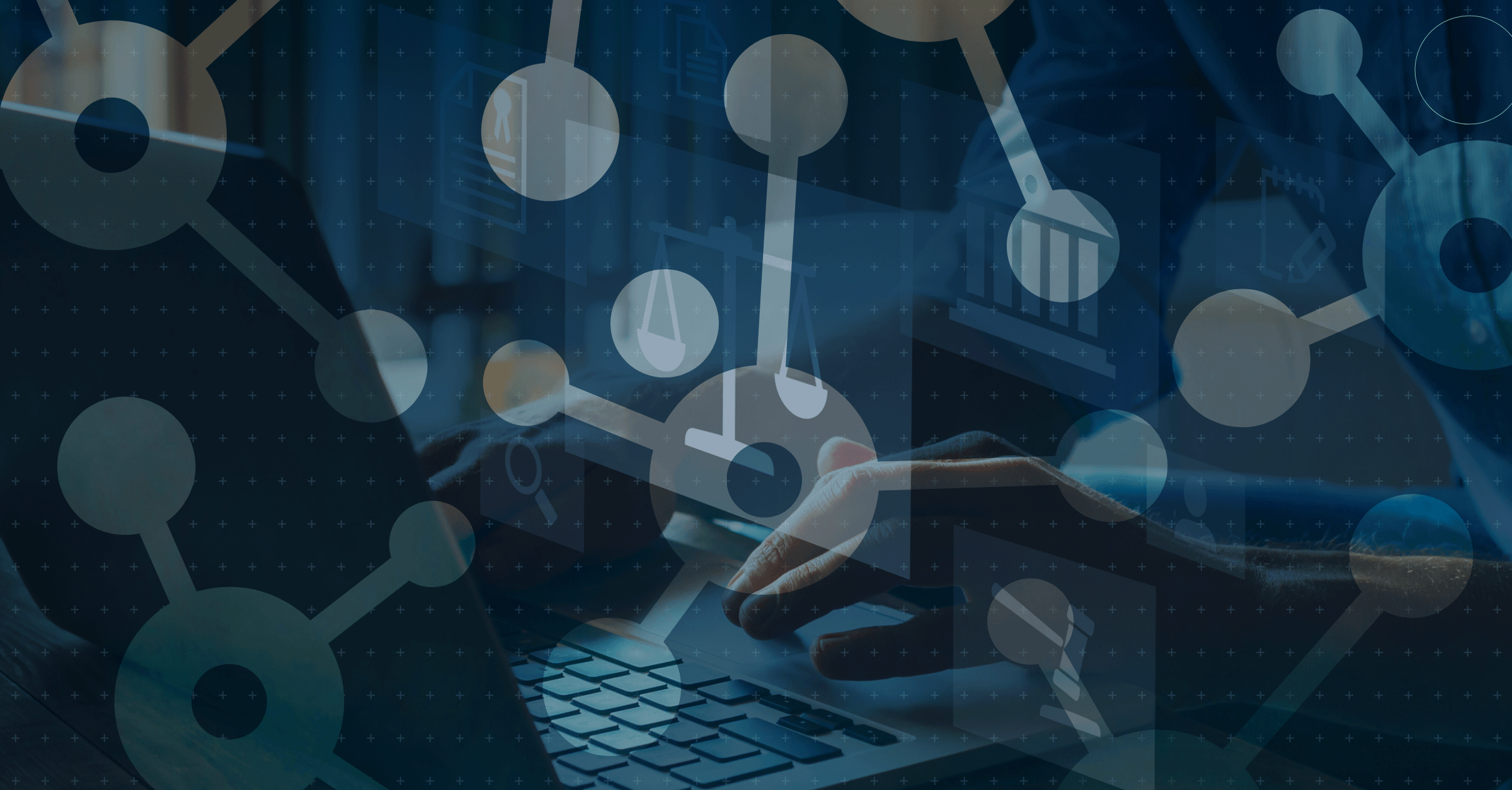
Visit Smart Counsel