This week, its more from the school of thought that ‘AI-regulation-based-on-existing-regulatory-principles’ would be a half-finished job. Last week we discussed a paper from Harvard’s Kennedy School on the need to radically rethink AI governance. This week we review a paper from Stanford’s Human-centered AI institute (HAI) about the shortcomings of current, long-held approaches to privacy regulation in an AI world:
..as we move toward a future in which AI development continues to increase demands for data, data protection regulation that at best maintains the status quo does not inspire confidence that the data rights we have will preserve our data privacy as the technology advances. In fact, we believe that continuing to build an AI ecosystem atop this foundation will jeopardize what little data privacy we have today.
Like the Kennedy paper, the HAI paper argues that the ‘individual harms and rights’ model which underpins current privacy laws fails to address the bigger, more complex societal risks posed by AI.
What’s wrong with current privacy models?
The HAI paper puts forward 4 ‘propositions and provocations’ in incrementally building up its argument for rethinking privacy in the AI era.
Proposition 1: Data is the foundation of AI systems, which will demand ever greater amounts of data
The paper starts with the unarguable proposition that to feed the insatiable appetite of AI, “[b]oth the totality of data, and the surface areas by which data is generated and collected, such as embedded sensors in household objects, smart appliances, and biometric cameras in public spaces, will continue to expand.”
The paper recognises that none of the AI advances achieved over the past decade would have happened without the largely unconstrainedavailability of massive amounts of data, combined with the more powerful computers, processing capacity, and cloud storage that developed at the same time. The paper also recognises that new extended AI privacy regulation of the kind the paper goes on to recommend could slow AI development, but that this would not be such a bad thing:
Some will respond..that adopting [our recommended privacy rules] will throw a wrench in the data economy and stall, if not destroy, progress in developing AI. We disagree. Pushing back against the status quo of ubiquitous data collection will not cripple data intensive industries. It might slow the rate of some progress in AI, though this may be a feature, not a bug.
Proposition 2: AI systems pose unique risks to both individual and societal privacy that require new approaches to regulation
AI exposes individuals to greater privacy risks compared to previous digital technologies by:
exacerbating existing privacy risks by further enabling what another commentator, Shoshana Zuboff, calls “surveillance capitalism,..[which] unilaterally claims human experience as free raw material for translation into behavioral data.”
creating a whole new set of risks, including such as new forms of identity-based risks, data aggregation and inference risks, personality and emotional state inferences via applications of phrenology and physiognomy, exposure of previously unavailable or redacted sensitive personal information, misidentification and defamation.
The HAI paper also argues that what marks AI out from previous generations of digital technology is that its potential privacy harms extend beyond the harms caused to an individual by misuse of their own personal information:
AI can “generate predictive or creative output that, through relational inferences, can even impact people whose data was not included in the training datasets or who may never have been users of the systems themselves.”
because AI operates at scale, AI threatens groups and society at large in ways that cannot be mitigated through the exercise of individual data rights. This capacity can be exercised to classify and apply outcomes “to large swaths of the population based on group affiliation— thereby amplifying social biases for particular groups.”
Proposition 3: Data protection principles in existing privacy laws will have an implicit, but limited, impact on AI development
The HAI paper acknowledges that, in theory, the two key requirements of most current privacy laws - minimising the data collected and only using the data for the purpose for which it is collected - should place constraints around AI development. However, the paper argues that these existing privacy restraints fall short in practice:
when dealing with the massive data requirements inherently required for most LLMs, it is difficult to define how much data is ‘too much’ data, and even if that was possible, the data pool would still be outsized compared to that stored and used by previous digital technologies.
“[a] core weakness with the current privacy framework is that individuals are assumed to have a level of control and power equal to that held by companies and institutions collecting and processing their data.” It is unrealistic to expect individuals to exercise these ‘privacy self-management’ rights “to prevent data collection in the first place in a society where it is difficult, if not impossible, for the majority of people to avoid interacting with technology” and when “the inequitable power dynamics of a data ecosystem in which the data collectors and processors, most of which are powerful private tech companies, [mean they] hold far more market power over personal data collection than do individuals.”
Proposition 4: The explicit algorithmic and AI-based provisions in existing laws do not sufficiently address privacy risks
The first wave of AI-specific privacy measures have tended to require that consumers be informed that automated decision making was being used, giving a right to opt-out, and that data protection impact assessments be undertaken, at least for high risk systems (e.g. the EU’s GDPR and now the new AI Act and the California Privacy Rights Act (CPRA).
The HAI paper gives the thumbs down to both measures:
while acknowledging that the opt-out right from automated decision making is a potentially powerful deterrent to its over-use, the underlying logic of this approach “doubles down on the privacy self-management approach, placing the burden on individuals to understand what automated decisionmaking is and why they may wish to opt out of it.” Also, most AI systems may escape these requirements because, strictly speaking, they are not used for decision-making purposes, “leaving open the question of whether they could be implemented in a way that consumers may not know that AI is being utilized but not subject to notice requirements.”
As regulators typically lack effective enforcement powers, data impact assessments are “are little more than a bureaucratic hurdle with no teeth” and the lack of industry or regulatory standards means there is a lot of variability in content and quality.
So, how should AI privacy be designed?
The HAI paper has three suggestions:
Suggestion 1: Denormalize data collection by default
The current approach underlying most privacy laws is to allow collection and use of personal data within the boundaries of the disclosed purpose, with the citizen having rights to view and correct the data and more recently a ‘right to be forgotten’: in effect, an opt out approach.
The HAI paper argues that while this opt out model may be legitimate for government data collection, for which the first privacy principles were originally developed, it was inappropriately carried over to private sector data collection:
The expansion of the FIPs from their original application to governmental data collection in the early 1970s to the private sector reinforced the approach of allowing data collection by default. There are legitimate reasons to allow governments to collect data in many circumstances without requiring individuals to give their explicit consent: tax collection, census taking, and provisioning public benefits are but a few examples. But applying this rationale to the private sector normalized the idea that individuals should have to opt out, rather than choose to opt in.
The paper acknowledges that opt-in would not be a simple to implement, and that the opt-in mechanisms themselves would require strict regulatory oversight to prevent technological avoidance: e.g. the use of dark patterns. However, the paper argues that, in the absence of such a fundamental shift in the data ecosystem, the pressing demands of AI’s data appetite would mean that “the incentives are such that companies will try to maximize data collection, especially if they are concerned their competitors will do so even if they do not.”
Suggestion 2: Focus on the AI data supply chain to improve privacy and data protection
A complex AI supply chain is emerging, with data being collected, stored and used in different ways along that supply chain, from training data sets used by the AI developers, finetuning foundational models to be domain-specific (e.g. for medical applications), supply of ‘AI in the cloud services’ and use of databases for retrieval-augmented generation (RAG) to tailor to enterprise-specific tasks.
The HAI paper expresses concern that there are ‘gaps’ in current privacy laws which mean that data collection and use in critical parts of the AI supply chain are not caught. In particular, privacy laws tend to focus on the personal information inputted by an individual user of AI and not on any personal data used at the AI development level:
data subjects are the individuals whose data was collected and included in the training data; model subjects are those subject to the decisions of the downstream model. Thus far, regulators have been focused more on the privacy issues raised for model subjects than data subjects, though the release of generative AI systems to the public surfaced the issue of what data protections applied to data subjects.The inclusion of personal or identifiable data in training datasets not only makes it possible that a model may memorize and output that data, it also raises the issue of consent. Do data subjects know that their data was included in a system’s training data? Were they asked for consent before being included? What rights do they have to request exclusion or deletion from these datasets? Can individuals have their personal data deleted from a model? And how do individuals even proceed in discovering whether their data was included?
The paper argues for regulatory measures including documenting the provenance or source of any data used for training and, if the data is related to an individual, include whether it was obtained with consent.
Suggestion 3: Flip the script on the management of personal data
The HAI paper calls for a fundamental realignment of the data supply ecosystem: “[n]early 30 years have elapsed since the creation of the commercial internet, and yet the fundamentals of online data exchange remain largely unchanged, specifically the unbalanced flow of data from consumers to companies.”
One of the suggested measures is to promote the emergence of data intermediaries who, by aggregating data from individuals as their customers, can provide the strength in numbers for individuals to negotiate in their best interests. However, the paper acknowledges that for data intermediaries to become a feature of the market there would need to be both regulatory change (such as a shift to opt-in) and technological developments, such as data portability.
Conclusion
We know two things: AI, with good guardrails, can be a ‘force for good’ and data is the ‘oxygen of AI’. This presents a challenge for policy makers, well described by the HAI paper:
The challenge moving forward in a world with greater demands for data is how to mitigate excess data collection without adding too much friction with excessive consent requests. Digital services need consumer data to operate, and not all such requests are excessive.
While the paper points to research which shows that LLMs can be built within the constraints of existing privacy principles of data minimisation and limited purpose, it is not clear what the impact on AI innovation would be from the more fundamental shifts it advocates. The paper candidly acknowledges that the EU’s requirements on ‘[c]ookie consents are a prime example of consent fatigue and how not to denormalize data collection” because it requires users to manage consent on a continual, site-by-site basis. The paper points to the better example of Apple’s rollout of app tracking transparency (ATT) in iOS 14.5, with which users are asked when they first open an app whether they wish to allow an app to track their activity across other apps and websites. The setting prohibits apps from using third party tracking methods unless the user approves it on a per-app basis.
But whether you agree or not with the HAI paper’s proposals to up-end current privacy law, it does make the good point, like the Kennedy School paper reviewed last week, that when thinking about safe and responsible AI an ‘individual harms and rights’ approach may not fully address the societal risks presented by AI.
The HAI paper was authored by Jennifer King and Caroline Meinhardt.
Read more: Rethinking Privacy in the AI Era
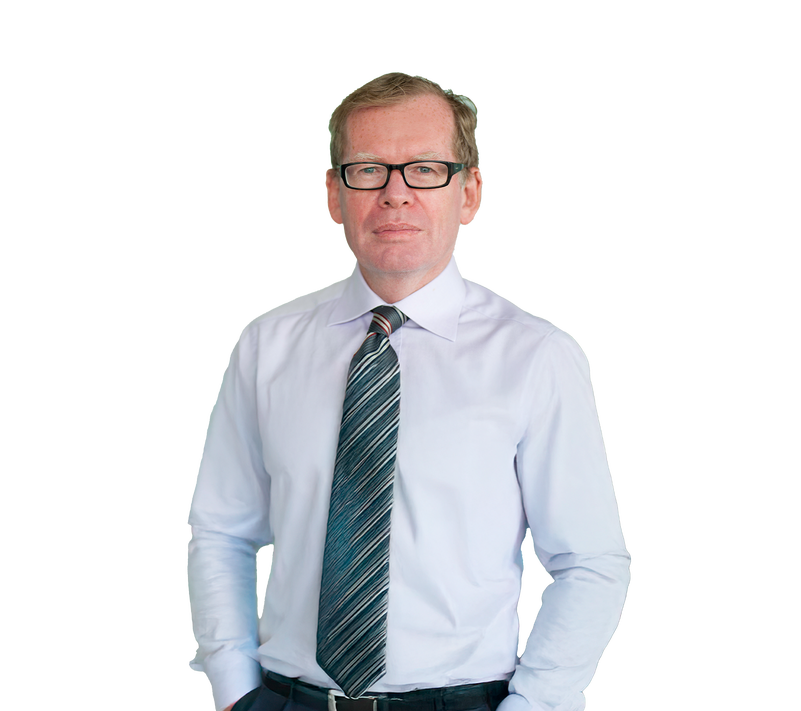
Peter Waters
Consultant