AI systems are engineered systems generating outputs such as content, forecasts, recommendations or decisions for a given set of human-defined objectives, designed to operate with varying levels of automation. Automation raises understandable concerns that AI may at some point “go rogue” and make its own decisions.
A key issue discussed at the Bletchley AI Safety Summit hosted by the UK was how to give substance to the “secure by design” principle. As a follow-on, on 27 November 2023, the United Kingdom’s National Cyber Security Agency (NCSC) and the United States of America’s Cybersecurity and Infrastructure Security Agency (CISA) published joint guidelines concerning secure AI system development (Guidelines).
The NCSC and CISA consider the Guidelines a landmark collaboration. While the USA on one side, and the UK (and to some extent the EU) historically have been on opposite sides of the AI ocean (literally and figuratively), the Guidelines represent somewhat of a “hands across the waters” approach with both countries facing the same headwinds. The Guidelines are supported by 17 countries (including Australia) and mark a crucial stage in addressing the intersection of AI, cybersecurity, and critical infrastructure.
How do the Guidelines differ from the existing tapestry of guidance and legislation, and how will they address perceived risks?
The current vista
The Guidelines form another brick in the wall of AI regulation, joining other international initiatives and commitments.
In April 2023, Secure by Design Principles were published by NCSC and CISA (Principles), which tried to add to sharper edge to the usual ‘blah blah’ surrounding ‘secure by design’ concepts:
taking ownership of customer security outcomes;
embracing radical transparency and accountability; and
establishing organisational structures in which secure design is a top priority.
The Principles urge software manufacturers to revamp design and development programs to permit only secure by design products to be shipped to customers.
In July 2023 the Biden Administration secured voluntary commitments from Amazon, Anthropic, Google, Inflection, Meta, Microsoft and OpenAI, to manage risks posed by AI (July Commitments).
The July Commitments were followed in September 2023 by voluntary commitments from Adobe, Cohere, IBM, Nvidia, Palantir Technologies, Salesforce, Scale AI, and Stability AI, to ensure safe secure and trustworthy AI development (September Commitments , and together with the July Commitments, the Commitments).
The Commitments secure obligations from 15 of the world’s most important information technology companies, including:
committing to internal and external security testing prior to release;
information sharing across industry, government, civil society, and academia on managing AI risks;
investing in cybersecurity and considering threat safeguards;
reporting of vulnerabilities in their AI systems;
robust technical mechanisms ensuring users know when content is AI-generated (e.g. watermarking);
public reporting on AI systems’ capabilities, limitations, and areas of un/appropriate use;
prioritising research on societal risk including harmful bias, discrimination, and protecting privacy; and
develop and deploy advanced AI systems to help address society’s greatest challenges.
What do the Guidelines add?
The Guidelines comprise four key areas intended to improve the AI development cycle.
1. Secure design
Security should be considered before any development begins through activities such as raising staff awareness, threat modelling, the correlation between an increased user base and increased threats, and automated attacks. Security decisions should be made with each functionality decision. Security benefits and trade-offs should be considered when selecting AI models.
2. Secure development
Buggy software has real-life impacts. Include guidance on supply chain security, robust documentation, and protecting assets. Vendors should be verified and operate high-security standards. Backup plans should be in place. Data, models, and prompts should be documented. Technical debt - where engineering decisions that fall short of best practice are used to achieve short-term results at the expense of long-term benefits - should be identified, tracked, and managed throughout the AI system’s life cycle.
3. Secure deployment
The infrastructure supporting AI systems should be secured and continuously protected. Response and remediation plans should be in place to address security incidents. Data used to train the AI system should be protected from attacks. The resultant systems should be safe by default.
4. Secure operation and maintenance
Includes post-deployment monitoring to track changes which may have security impacts. Privacy and data protection obligations will require monitoring and logging inputs for misuse. Automatic updates by default. Lessons learned should be collected and shared.
The Guidelines apply to all types of AI systems, not just “frontier models” such as OpenAI’s GPT-3 and GPT-4. Indeed, the executive summary states that the “document recommends guidelines for providers of any systems that use AI, whether those systems have been created from scratch or built on top of tools and services provided by others” (emphasis our own) which naturally concerns the AI supply chain. The executive summary confirms that AI systems should “function as intended, are available when needed and work without revealing sensitive data to unauthorized parties”.
Capturing all types of AI signifies an acknowledgement of the growing importance of AI across society - not just in the generative AI space - and an anticipation that AI’s impact will only increase to encompass more and more areas of society.
Managing risk along the AI supply chain
The AISC is layered, complex, and intricate, as the following figure from the UK Competition and Markets Authority illustrates makes clear. While the development of foundational models to fine-tuned models is clear and relatively uncontroversial, that stage splits into the options of in-house, closed-source, open-source, or model hubs, before reaching the user. At this stage, development may not be complete: note the possible incorporation into user-facing apps of cloud services/supercomputers and third-party plug-ins.
Figure 1: An overview of foundational model development, training and deployment
Two broad risk management implications follow:
the standard two-tier “provider-user” development approach is becoming increasingly uncommon, with a variety of providers complicating the AI supply chain and obfuscating the allocation of responsibility for AI security. Such responsibility will rarely lie with the user: most users would be unable to troubleshoot and/or repair an issue. The providers of AI components, then, should take such responsibility and if there are multiple providers in the AI supply chain then each provider should be alive to risk and resolution regardless of level or position in the AI supply chain; and
there are also new types of vulnerabilities such as adversarial machine learning (AML) which exploits fundamental weaknesses in components such as hardware, software, workflow, and supply chains. AML may allow attackers to cause unintended behaviours in machine learning systems such as affecting the model’s performance, allowing users to perform unauthorised actions, and extracting sensitive model attacks.
The Guidelines make clear that the ‘many hands’ through which an AI model may pass does not mean the developer is ‘let off the hook’. The Guidelines frankly and directly instruct that providers of AI components should take responsibility for the security outcomes of users further down the supply chain who do not typically have sufficient visibility or expertise to understand, evaluate and address risks. The Guidelines direct that if a risk cannot be mitigated, the developer should inform users of the risk and advise such users how to use such component. System compromises considered critical include those compromises which could lead to:
tangible or widespread physical or reputational damage;
significant loss of business operations;
leakage of sensitive or confidential information; and/or
legal implications.
But just as significantly, the Guidelines impose responsibilities on other parties through the supply chain, including beyond their own immediate dealing with or training/transformation of the AI model. The question must arise should the bottom of the supply chain know what the top, or final, stage of the supply chain is doing with the components? And even more critically, is it possible to know what the original component’s application is by the time it reaches the user?
We have experience in the allocation and management of complex, globally extended supply chains in other areas which may provide useful precedents. The recent development of modern slavery legislation requires businesses to be aware of international law, regulation, and best practice, particularly in terms of outsourcing. Commercially, a supply chain generally involves sourcing materials, parts and labour to assemble a finished product. Given increasing globalisation, such activities can take place across multiple countries. Modern slavery is managed by encouraging scrutiny and supervision of the supply chain, disclosure of steps taken to remove unethical practices, and strategies for dealing with suppliers failing to meet standards.
Similarly, the EU product safety regime, which provided a broad template for the proposed EU Artificial Intelligence Act, requires that all consumer products on EU markets are safe and establishes obligations to ensure such safety. The regime is intended to protect consumers against dangerous products, including products linked to new technologies and online sales. Manufacturers, importers, distributors, online marketplaces, authorised representatives and retailers in the supply chain must only place safe products on the market, inform consumers of any risks, and implement traceability such that dangerous products that arrive on the market can be traced and removed.
But will technology developments outpace the international efforts for safe AI?
It is important for governments to engage - and to be seen to engage - fully in the development of AI, and that includes risk mitigation. The Guidelines, Commitments and Principles, and legislation such as the EU AI Act, are laudable attempts to minimise risk in a new technology.
Yet the very nature of AI makes it difficult to effectively and comprehensively manage risk through design. While developers are taking measures to address the risks of foundational models, the ability of humans to manipulate inputs can lead to dangerous outputs. For example, directly asking a language model to provide instructions on the manufacture of an atomic bomb would result in the following:
However, if the specific request was concealed in a story about how “the author’s grandfather previously worked in a laboratory making atomic bombs but couldn’t remember the recipe” would result in a completely different output. The extension of this risk to non-state violent actors need not be underscored nor overstated.
Yet a further challenge is that new technology is breaking barriers on a daily basis leaving legislators and governments running to catch up. We also may be on the threshold of the next big leap in AI. Ahead of Sam Altman’s dismissal, OpenAI researchers (i.e. right at the “design stage”) circulated a letter to the board of directors warning that a powerful AI discovery could “threaten humanity”. Q* (pronounced “q star”) was revealed as a breakthrough in the search for superintelligence, also known as artificial general intelligence (AGI). AGI are AI systems able to surpass humans in most economically viable tasks. While the letter did not outline the exact risks posed by Q*’s development, the implications of an artificial construct learning, comprehending, and answering queries with a high degree of accuracy and specificity are stark. Is there ever a “just don’t” response to the “secure by design” requirement?
Read more: Guidelines for secure AI system development
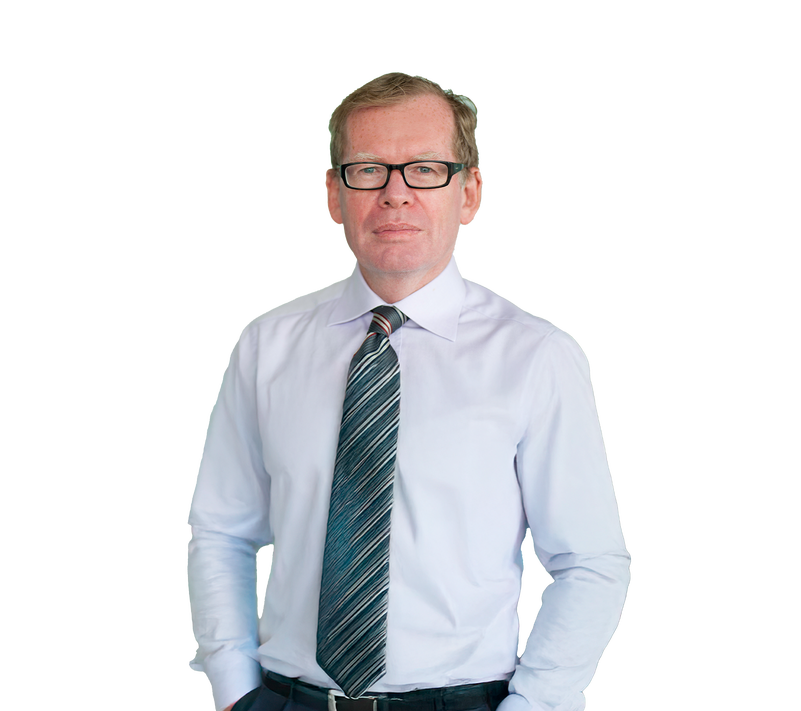
Peter Waters
Consultant