Just before Christmas, the European Data Protection Board (EDPB) released an opinion on how AI can be developed, trained, fine-tuned and used consistently with the EU’s General Data Protection Regulation (GDPR). Given the GDPR’s global reach, the EDPB’s opinion could impact AI globally.
When is an AI model anonymous?
The GDPR does not apply to use of anonymous information: defined as information which is not related to an identified or identifiable natural person, taking into account ‘all the means reasonably likely to be used’ by the data controller or another person.
Typically, AI models are trained with personal data to make inferences about individuals different from those whose personal data was used to train the AI model. Once the correlations between the data on the mass of individuals is encoded as parameters, the training data is discarded from the AI model. For example, an AI radiological tool trained on historical breast screenings of hundreds of thousands of women will be fed the scans from a new patient.
While that might seem like a good start to satisfying an anonymisation standard, the EDPB concludes otherwise because:
..information from the training dataset, including personal data, may still remain ‘absorbed’ in the parameters of the model, namely represented through mathematical objects. They may differ from the original training data points, but may still retain the original information of those data, which may ultimately be extractable or otherwise obtained, directly or indirectly, from the model.
The EDPB set a high threshold for an AI model to be treated as anonymous:
With earlier generations of digital technology, pseudonymisation of data (for example masking names and other identifying information) before feeding the data into the processor was usually sufficient. However, given that the central function of an AI model is to find correlations between apparently unrelated data, it may be possible to extract accurate or inaccurate (because statistically inferred) personal data from an AI model, even though the training data was fed into the model and encoded “in a way which that does not make the relation with that natural person immediately apparent”.
In evaluating whether anonymity could be unravelled taking into account all the means reasonably likely to be used by the controller or other person, the data protection regulator’s assessment “might differ between a publicly available AI model, which is accessible to an unknown number of people with an unknown range of methods to try and extract personal data and an internal AI model only accessible to employees”. Developers will need to test a publicly available AI model’s resistance to:
Attribute and membership inference (that is that a person was a member of the training data cohort).
Exfiltration (data extraction by a hacker).
Regurgitation of training data and model inversion (using outputs to infer the model’s parameters).
Reconstruction attacks (partially rebuilding the training data set), all from multiple possible use and attack vectors.
If the developer has not thoroughly documented each stage of AI model development and training, its decisions about whether to use personal data rather than alternative data sources, whether to anonymise the data sources, how to mitigate identification risks and whether those safeguards can withstand testing, a regulator is entitled to conclude that effective measures were not taken to anonymise the AI model.
Citing an EU court decision, the likelihood of obtaining, intentionally or not, such personal data from queries, should be insignificant for any data subject.
Legitimate purpose
The GDPR permits use without consent of personal data by a controller where necessary for the legitimate purposes of the controller or a third party. This requires three conditions to be met:
The pursuit of a legitimate interest by the controller or by a third party.
The processing is necessary to pursue the legitimate interest.
The legitimate interest is not overridden by the interests or fundamental rights and freedoms of the data subjects.
The EDPB gives the following examples of the first condition (legitimate purpose) when applied to AI:
Developing the service of a conversational agent to assist customers of a business.
Developing an AI system to detect fraudulent content or behaviour.
Improving threat detection in an information system.
The EDPB says that applying the second condition (necessity) to AI requires asking whether:
The purpose could be achieved using non-AI technology.
The purpose could be achieved using AI not trained on personal data.
The amount of personal data used in training is proportionate to the benefits and value of the purpose.
The third condition (balancing legitimate purpose with fundamental rights) is the most difficult to apply – and satisfy – for AI trained on personal data. The EDPB identifies the following considerations:
Data subjects’ interests extend beyond their privacy to include an interest in self-determination and retaining control over one’s own personal data.
Broader social impacts need to be weighed in the balance. Large-scale, indiscriminate data collection by AI models in the development phase may create a sense of surveillance, leading individuals to self-censor, and present risks of undermining their freedom of expression. In the deployment stage, there also may be risks to freedom of expression from AI blocking content, to mental health by AI serving offensive or distressing content to vulnerable users, or to fairness in labour markets from discriminatory AI.
Although strictly speaking consent is not required for legitimate purpose, the reasonable expectations of data subjects about use of their data in training AI are still relevant. As AI is complex and there are multiple potential uses of personal data in the AI supply chain, the information which is provided to data subjects by AI developers and deployers can help shape user expectations. However, the EDPB cautions while the omission of information can contribute to the data subjects not expecting use of their data, the mere fulfilment of the GDPR transparency requirements, such as in terms and conditions, is not sufficient to consider the data subjects can reasonably expect a certain processing.
Against the above downsides of using personal data in training AI must be weighed against the benefits of a better trained AI, for individuals and society.
The balance between legitimate purpose and fundamental rights needs to be separately assessed for the data subjects whose personal information is used in training an AI model and then for the data subjects whose personal information is analysed by the trained model once deployed.
A developer or deployer is more likely to be able to satisfy the balancing test where there is a direct relationship between the developer or deployer and the data subject whose personal data is being used. This relationship both makes it more practicable to fully inform the data subject of the use of their data in the AI model and the data subject is more likely to be aware that his or her data will be used to ensure a more personalised service is delivered using the AI. By contrast, the EDPB is particularly scathing of web scraping in the development phase because it “may lead – in the absence of sufficient safeguards – to significant impacts on individuals, due to the large volume of data collected, the large number of data subjects and the indiscriminate collection of personal data”.
A developer or deployer can add some credits on its side of the balance by adopting mitigation measures. These could be built-in technical guardrails limiting deepfakes or misinformation. In some incentive regulation, the EDPB also encourages developers and deployers to consider consumer protection measures which go beyond EU law, including by expanding opt out rights, broadening the ‘right to be forgotten’ beyond the specific GDPR grounds, allowing data subjects to submit claims of personal data regurgitation or memorisation so that unlearning techniques can be improved, providing more transparency than required by providing additional details about the collection criteria and all data sets used and publishing a frank statement of their reasoning in balancing legitimate purpose with fundamental rights.
Unhelpfully, the EDPB provides no real guidance on how this list of disparate, qualitative considerations are to be weighed individually and against each other. Clearly, this third condition constrains the scope of ‘legitimate purpose’ in the use of personal data in training and deploying AI.
Mario, is anyone listening?
In his report on European competitiveness, Mario Draghi cautioned the net effect of the gathering mountain of EU AI regulation, while well-intended to protect consumers and citizens, "is that only larger companies – which are often non-EU based – have the financial capacity and incentive to bear the costs of complying. Young innovative tech companies may choose not to operate in the EU at all”.
This opinion from the EDBP seems more of the same.
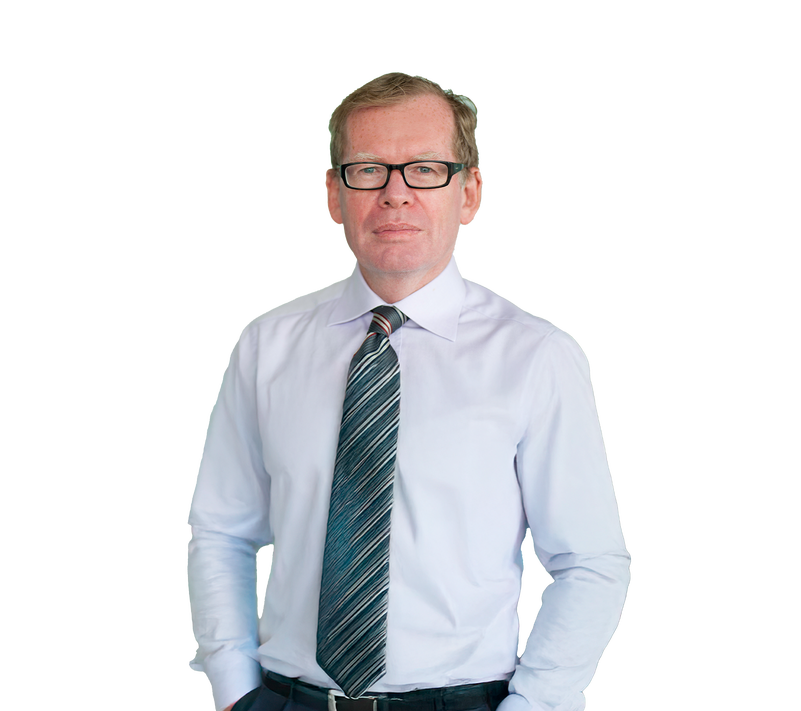
Peter Waters
Consultant