Stanford University’s Human-Centered Artificial Intelligence (HAI) has recently released its annual global AI Index surveying the trends in AI research, ethics, investment and commercial use. As it is a thumping 384 pages, we will review the 2023 AI Index in instalments over the next few weeks. This week we look at technological developments - essentially ‘how good has AI really become’?
Who’s doing the R&D on AI?
HAI’s primary conclusion is that there has been a seismic shift in AI research from the university sector to the private sector. Until 2014, most machine learning systems were released by academia. In 2022, there were 32 significant commercially produced machine learning systems compared to just three produced by academia.
The ‘privatisation’ of AI is likely to be irreversible because, as HAI notes, “[b]uilding state-of-the-art AI systems increasingly requires large amounts of data, computer power, and money—resources that industry actors inherently possess in greater amounts compared to nonprofits and academia.” For example, PaLM, launched by Google in 2022, had 540 billion parameters, nearly 360 times more than GPT-2 released in 2019, and PaLM cost US$8.1 million to train compared to US$50,000 for GPT-2.
Unsurprisingly, the development focus is on large language models (LLMs), like ChatGPT. Of the 36 significant AI machine learning systems released in 2022, LLMs were the most common class of system: 23 significant LLMs were released, roughly six times the number of the next most common system type, multimodal systems, with only 2 new specialist vision and 2 new speech systems.
China outstrips the rest of the world for research on AI. Nine of the top 10 institutions with the highest output of AI articles are Chinese (with MIT languishing at number 10). Chinese authors accounted for nearly 40% of AI publications in 2022.
Yet of those 36 significant machine learning systems released in 2022, 16 were from the US, 8 from the UK (suggesting some success in the UK Government’s strenuous efforts to promote AI), 3 from the EU (suggesting that the EC’s equally as strenuous efforts to promote AI are yet to bear much fruit), and only 3 were from China.
Yet this is not to under-rate the Chinese-developed AI models:
GLM-130B is a bilingual English and Chinese LLM , created by researchers at Tsinghua University, is described as “impressive” by HAI and others say is better than OpenAI’s GPT-3 and Google’s PaLM.
In medical image segmentation, AI systems segment objects such as lesions or organs in medical images, and performance is measured in mean Dice, which represents the degree to which the polyp segments identified by AI systems overlap with the actual polyp segments. The top-performing model in 2022 was created by a Chinese researcher with a mean Dice of 94.1%.
As an apparent counterpoint to ‘AI nationalism’, HAI also emphasised the importance of cross country collaboration on AI: “[c]ross-border collaborations between academics, researchers, industry experts, and others are a key component of modern STEM (science, technology, engineering, and mathematics) development that accelerate the dissemination of new ideas and the growth of research teams.”
As depicted in the figure below, most cross country collaboration is between US and Chinese researchers, but possibly reflecting geopolitical headwinds, the total number of U.S.-China collaborations only increased by 2.1% from 2020 to 2021, the smallest year-over-year growth rate since 2010.
Australian collaborations separately with Chinese researchers and with US researchers are amongst the most prevalent globally, suggesting that Australia’s AI researchers may be both punching above their weight and successfully navigating those geopolitical headwinds.
Just how good is AI?
While there has been much hoopla around what generative AI models such as ChatGPT can do, HAI has a more sober assessment of the progress of AI capability in 2022: “AI continued to post state-of-the-art results, but year-over-year improvement on many benchmarks continues to be marginal.”
HAI uses a range of task-related benchmarks to test AI’s performance, and as the following diagram shows, while there have been very big increases in performance since the benchmarks were first used on AI (the blue part of the bar), the YoY improvement in 2022 was very incremental (the purple part of the bar): for all but 7 of the benchmarks, the improvement registered is less than 5%.
To take a tangible example, in 2020, the top result for speech recognition AI (words to text) was posted by American researchers, whose model achieved an equal error rate of 0.1%. But that represents only a 0.28 percentage point decrease on the error rate compared to the state-of-the-art result achieved by Chinese researchers in the previous year.
Does this mean AI has hit a developmental ‘brick wall’? Yes and no - there appear to be 4 different things going.
Practice makes perfect
On the positive side for AI, HAI says that “the speed at which benchmark saturation is being reached is increasing.” This means that AI performance against some benchmarks has now become so good - so close to a perfect score - that inevitably any further improvement is incremental, if not vanishingly small.
For example, HAI tracks progress of facial recognition AI through the US National Institute of Standards and Technology’s Face Recognition Vendor Test, which tests how well different facial recognition algorithms perform on various homeland security tasks, such as identification of child trafficking victims and cross-verification of visa images. Facial detection capacity is measured by the false non-match rate (FNMR). As of 2022, the top-performing models posted an error rate below 1%, and as low as 0.06% on some data sets. Improved accuracy alone is not, of course, a basis for use of FRT given the larger privacy and equity considerations around its use.
AI has outgrown some benchmarks
Again on the positive side for AI, HAI notes many existing language benchmarks do not accurately test how capable language models are at applying the knowledge they learn across different domains. This criticism is particularly apposite to the new generation of generative AI models, which can be used across many different sectors, from medical diagnosis to insurance claim processing to writing university essays.
HAI therefore uses a benchmark called Massive Multitask Language Understanding (MMLU), which evaluates models in zero-shot or few-shot settings across 57 diverse subjects in the humanities, STEM, and the social sciences.
HAI found that Gopher, Chinchilla, and variants of PaLM have each posted state-of-the-art results on MMLU, and the pace of YoY improvement is amongst the fastest across the HAI benchmarks.
AI struggles with reasoning
On the negative side for AI, HAI observes that while “[l]anguage models continued to improve their generative capabilities, new research suggests that they still struggle with complex planning tasks.” Or as Yann LeCun, Facebook’s chief AI scientist puts it more colourfully, AI has less common sense than a domestic cat.
This can be illustrated by comparing two benchmarks from the 2023 AI Index report.
The first benchmark is the Visual Question Answering Challenge (VQA) which tests AI systems with open-ended textual questions about images: for example as depicted below:
The top-performing model was PaLI, a multimodal model produced by Google researchers, which scored better than the human benchmark as depicted below:
The second benchmark is Visual Commonsense Reasoning (VCR), which is a relatively new benchmark in which AI systems must answer questions presented from images, as in VQA, but the AI has to go further to select the reasoning behind their answer choices.
As the figure below shows, AI systems consistently perform well below human benchmark levels on this more complex reasoning test. HAI notes that “VCR is one of the few visual benchmarks considered in this report on which AI systems have yet to surpass human performance.”
The next two instalments will look at the 2023 AI Index’s data on investment and commercial use of AI and AI ethics.
AI struggles to understand us
And lastly again on the negative side for AI, it struggles to accurately identify human emotions.
Sentiment analysis AI applies language techniques to identify the sentiment of a particular text. It is used by many businesses to better understand customer reviews.
The Stanford Sentiment Treebank (SST) is a dataset of 11,855 single sentences taken from movie reviews that are then transformed into 215,154 unique phrases whose sentiments have been annotated by human judges.
In 2022, AI posted a new high score on the SST benchmark - but that was only 59.8% posted by the Heinsen Routing + RoBERTa Large model, and the YoY is looking like it will plateau.
AI as the new ‘Q’
A striking development identified by HAI was that the AI models are starting to rapidly accelerate scientific progress. Examples given by HAI include:
nuclear fusion, which promises safer, cleaner nuclear energy, is achieved using a machine called a tokamak, which controls and contains the heated hydrogen plasma. The problem is that the plasmas produced in these machines are unstable and necessitate constant monitoring. In 2022, researchers at DeepMind developed a reinforcement learning algorithm to discover optimal tokamak management procedures.
Antibody discovery using traditional means typically requires immense amounts of time and resources, often resulting in antibodies which are suboptimal (requiring the researchers to go back to scratch over and over again). Generative AI has been used to create antibodies in a zero-shot fashion, where antibodies are created with one round of model generation without further optimization. These AI-generated antibodies are also robust. This AI-based approach should substantially accelerate drug discovery.
HAI also identified ‘self generating AI’ as another emerging trend. Nvidia, a chip maker, used AI to design a chip which is 25% smaller but as fast and functional as the chips it has previously designed using standard electronic design automation tools.
Read more: The AI Index Report: Measuring trends in Artificial Intelligence
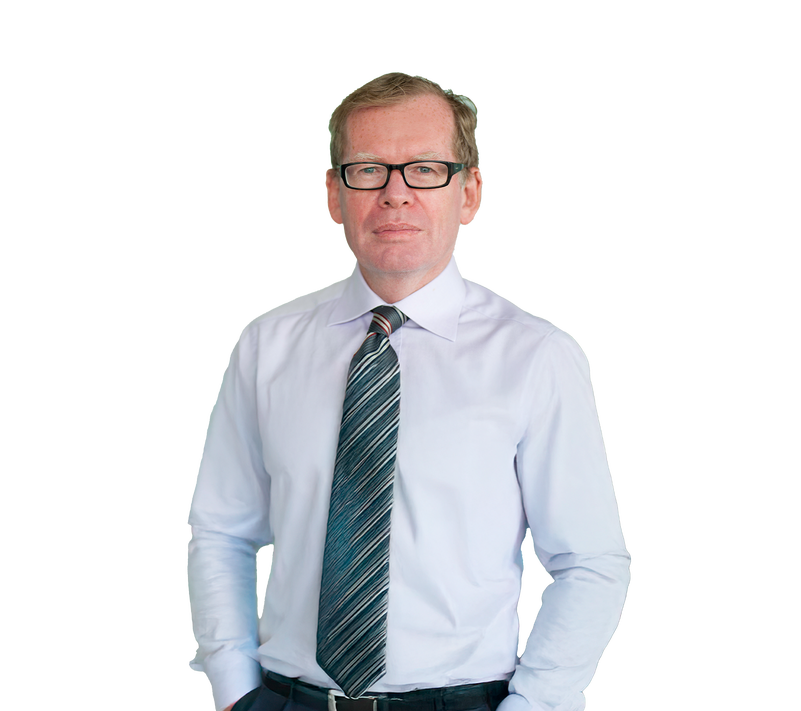
Peter Waters
Consultant