A future where the health system is increasing driven by digital technologies inevitably requires the collection and use of large quantities of highly personal data about users of the health service. However, a recent study by the UK’s Ida Lovelace Institute and the Health Foundation has found that health AI can systemise health inequities because the socially disadvantaged are ‘invisible’ to the algorithm:
‘there are limitations to the use of an algorithm-driven approach for identifying the clinically extremely vulnerable population which were exacerbated by poor availability of high-quality data. [and] not all clinically extremely vulnerable individuals were identified through the centrally developed algorithm due to lack of linked data or incomplete medical records, and many people would have been missed had they not been added to the shielded patient list by local clinicians.’
The case for health AI to capture social disadvantage
The COVID-19 pandemic threw into sharp relief the impact of social determinants of health: those younger than 65 in the poorest 10% of areas in England were almost four times more likely to die from COVID-19 than those in the richest; in Australia it was almost as bad, with the poor were three times more likely to die of COVID . The Health Foundation commented that::
“While broad data insights had suggested ethnicity links to health outcomes, what was clear was that, genetics-related or not, the pandemic’s impacts had deep rooted links to historical and institutionally ingrained cultural practices, which persistently left the same groups marginalised and facing increasing negative outcomes.”
The non-medical factors influencing an individual’s health are depicted as follows:
This, of course, represents a vast amount of information; separately collected cross a wide range of clinicians, laboratories, private and public service providers, charitable institutions and government agencies; and stored and formatted in a myriad of different systems: My Health Record illustrates just how difficult this can be for traditional data processing technology. But ingesting and analysing vast pools of data to find relationships and patterns is exactly what AI is all about.
The Ida Lovelace study, using COVID as a case study, set about identifying the reasons why important information about social disadvantage was not making its way to the NHS AI platforms - what the study described as ‘knots in the data pipeline’.
The knotted data pipeline
The study found that people involved in building data-driven systems can have a poor understanding of health inequalities, which might seem surprising in a universal public health system like the NHS whose foundational value is to ensure equal access in health services.The study pin-pointed a number of causes:
inequalities were not regarded as clinical safety concerns: this seems a polite way of saying that health professionals were too narrowly focused on the clinical presentation.
technology manufacturers and deployers pushed instead for risk assessments that were not informed by an equity outcome: this seems a polite way of saying that commercial developers did not themselves have a strong understanding of or perhaps even care much about social inequities.
No clarity about who is responsible in the health bureaucracy for directing and reminding those designing and deploying tools to ensure their tools worked for the populations they were looking after: this sounds a little like the evidence at the Robodebt Royal Commission.
trade-offs between different stakeholders within the NHS, such as privacy considerations.
when inequalities were considered, they were too broad-brush, only including ‘regular’ issues such as language, ethnicity and sometimes accessibility, missing more granular information on a person’s individual socio-economic situation.
The study called for a ‘humanities-based agenda in health, which will help interrogate ways in which inequalities are observed and mitigated in data-driven systems.’
In a pretty blunt broadside at centralised IT planning and politicians managing health systems, the study said that:
Differing approaches to design and delivery of data-driven systems at the national and local level are linked to political priorities and power dynamics, which can compromise the quality of insights.These power dynamics and ways of working often bypassed or obscured local intelligence and conversations which can flag demographic information that is important for mitigating inequalities. Ultimately, deciding what was commissioned became a political issue, and there is no evidence that anybody took responsibility for adapting the commissions to what emerged as the neediest areas.
The old ‘garbage in, garbage out’ problem is particularly damaging in health related systems because any interventions (especially in a health emergency like a pandemic) made based on poor quality data are at risk of being inaccurately targeted, misaligned with the situation on the ground, and disadvantaging people who aren’t represented in the data.
Data problems found by the study included:
missing data on people in the data pool. For example, when ethnicity data is missing for patients, coverage of certain demographics becomes patchy. When emerging insights are based on this incomplete data, it can lead to worse outcomes for those demographics.
people missing from the data pool. Political decisions can mean that access to health services can be restricted, such as people seeking asylum.
time-delayed or out-of-date data was also a problem, particularly in the context of a quickly evolving pandemic situation where resources, such as vaccines, are being allocated on a close to real time basis.
The study recommended that, given the breadth of data needed to be captures, deploying a diverse and multi-disciplinary team to data collection activities gives higher chances of picking up gaps in data and ensuring the pipeline does not lose vital contextual information.
The study expressed concern that when AI and data processing tools are focused on fast data availability and seamless data flows, the lived experience of potential patients gets ‘flattened out’ - usually with the granular information on social determinants of health being lost in the aggregation process.
The study recommended that:
local providers should have and manage their own raw data, instead of having data insights imposed on them by various other platforms through centralised curation and storage.
While some degree of data centralisation and standardisation was needed in a nation-wide system like the NHS, system engineers and product designers need to think about what might be lost in standardisation and visualisation of data, and ways to embed flexibility in platforms, for example allowing inclusion of context-specific information.
The study notes that there is a risk that data can be taken to present a complete version of truth or reality, whereas “there are always limitations to what data can tell us, and trusting too much in data as a proxy for reality could lead to poor quality insights”. As one participant in the study remarked:
‘Dashboards can be seductive, give you nice clean view of the world and you forget it’s just a representation of the world that may be skewed. Part of our job is to tell you why it’s skewed, and that’s a constant challenge.’
A common data processing response to gaps in ‘real life’ data is to use proxy data. While using proxy data can help to infer or estimate something not covered in a dataset, the study cautioned “this isn’t a silver bullet, and it’s still important to look for where data diverges from reality.” The study cited the example of a team which turned to prescription data to give them an idea of what conditions people in the datasets they were analysing might have been experiencing (since GP recording of health conditions was not always updated). These team itself was clear that, while this might have been a workaround for missing data, it still left questions around accuracy because a lot of the medication could be prescribed across different health conditions.
The study considered that:
having more granularity and context on data was helpful but importantly, the ability for data users to interrogate and be in discussion with data curators, data generators and platform owners was even more valuable as it helped to unpick some of the knots in the data pipeline. This interdisciplinary approach enabled more accurate and specific insights, (and in turn, more appropriate interventions in a particular area) than would have been achieved by using one general dataset.
During the pandemic, the UK Government used statutory mechanisms to allow pooling of multiple separate data repositories which would not have been possible under privacy laws. Study participants expressed concern that about how data-driven systems would be less able to tackle inequalities if these data channels were to close now the pandemic was fading. In its policy statement Data Saves Lives, the UK Government has said it will continue to allow data sharing, but the dimensions of this are not clear.
The study also cautioned that improved health risk assessment through data analysis needs to be accompanied by the wrap-around services that support the people who have been identified as at risk. The UK’s expanded COVID data analysis led to an extra 1.7 million clinically vulnerable people being (rightly) advised to stay at home a year into the pandemic, but this had implications for many because accompanying economic welfare support was not always considered or provided for as the pandemic wore on.
The study said that analysing data without contextual knowledge can lead to misunderstandings and poor-quality insights.
The study gave an example using the R number for COVID (we should all remember from those COVID press conferences that R is the number of people that one infected person will pass on a virus to, on average). In areas where there was relatively good health but a transient or low-paid population, R numbers might not have had as large an impact on the overall health of the population as in those areas with relatively worse health and the same employment status. If health resources were crudely allocated or infection control measures were based on the R number, the sub-set of migrant workers in the relatively well-off area have to decide between putting themselves at risk of infection (by going out to work) or staying at home and with limited public health or other support because it has been directed elsewhere.
The study recommended partnerships with local organisations, councils and charities and use of multi-disciplinary teams to give this vital context to health data. The study gave an example where a local public health team had combined about 30 databases including utility, social housing, benefits claimants and safeguarding databases, to create a combined inequality score with which they stratified their population.
Conclusion
The study concludes that the approaches which are likely to have the most impact on these knots in the health data pipeline are:
those that design local interventions and tools for people’s experiences of health and wellbeing inequalities. This would create a critical mass of knowledge about what the inequalities we’re interested in addressing are, what metrics to measure successes by and how data can relate to inequalities (e.g. intersectionality).
those that take on wider systems-thinking decisions and information flows within the NHS databases, to create a combined inequality score with which they stratified their population. This will result in practitioners realising their roles within the data pipeline and shaping the ways decision-making influences outcomes.
The study’s key message is that we need to “move away from seeing data as simply categorisations of people, for instance, and instead embed the data within dynamic and complex contextual frameworks that encompass the experiences of people and the roles of institutions and those who work in them.”
Privacy issues do not get much of a guernsey in the Ida Lovelace study. Maybe this is because the UK’s National Data Guardian has found that there was a high level of public support for health and social care data being used for public benefit (implicitly without individual patient consent). There is probably something of a virtuous circle here: the impact of data-driven systems’ ability to address health inequalities can reinforce the public’s trust that the health service is being true to its mission of fair and equal access, and in turn, this can reinforce the public’s support for the pooling of health and social care data to identify those social inequities in the first place.
Read more: A knotted pipeline | Data-driven systems and inequalities in health and social care
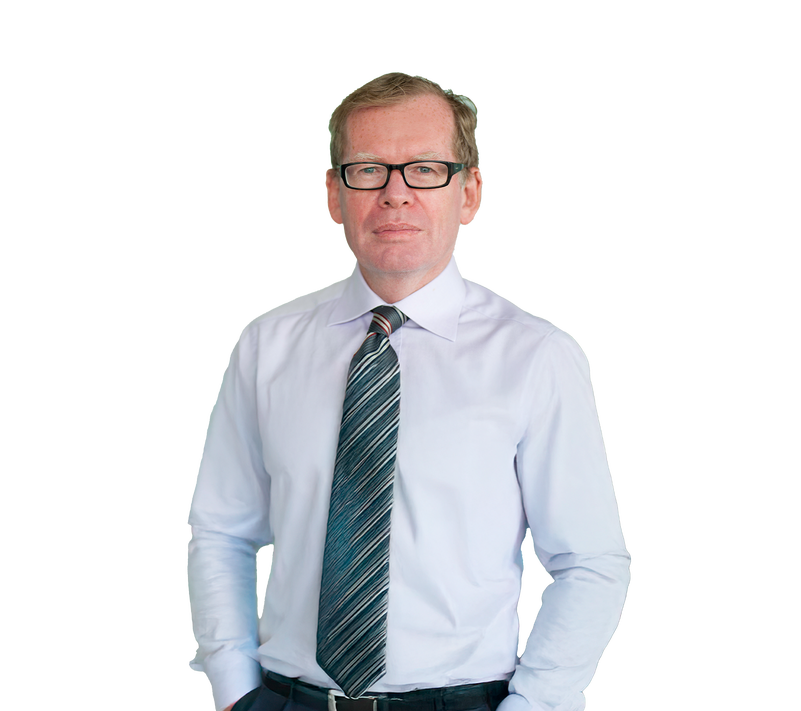
Peter Waters
Consultant