The EU has released a draft Code designed to provide guidance for providers of general-purpose AI models (GPAI) and the more strictly regulated GPAI with systemic risk on how to comply with the new AI Act. The Code was developed in collaboration with hundreds of stakeholders across industry, academia and civil society and after receipt of 430 submissions.
The Code is likely to set standards globally. Two of the chairs of the Code working groups (Yoshua Bengio, one of the godfathers of AI, and Nuria Oliver who holds many global AI governance positions) have said:
The Code also has global significance: it is the first time that legal rules are turned into more detailed guidelines for the responsible development and deployment of GPAI.
The Code of Practice for general-purpose AI offers a unique opportunity for the EU.
Transparency is key
The draft Code’s extensive disclosure obligations serve three objectives:
Give the new EU regulator, the AI Office “sufficient visibility into trends in the development and deployment of general-purpose AI models, particularly of the most advanced models”. This allows an agile regulatory approach as the future capabilities and directions of AI unfold.
Recognise the responsibility of AI providers to inform the AI value chain, given their GPAI models may form of downstream applications.
Protect artists, authors and other creators in the way content is created, distributed, used and consumed by GPAI.
Disclosure of how the AI model works
The draft Code sets a baseline of information to be disclosed by the developer about its GPAI:
Description of the intended and restricted tasks and of the downstream systems into which the model can be integrated, including high-risk AI applications.
Documentation about how the model interacts with hardware and software.
Description of the model architecture, the total number of parameters, the number of parameters that are active during inference, and context limits. The AI Office must be given greater detail, including the number of layers in the model.
Core elements of model training (for example, training stages, the objectives being optimised, the methods of optimisation, constraints), not only the how but also the why. The AI Office also must be given test results and a description of the computational resources used and energy consumed.
Data acquisition methods (for example, web crawling, data licencing, data annotation, synthetically generated data, user data), details about the data processing (if and how harmful or private data are filtered) and which data is used at each stage of training, testing and validating the model.
Protecting copyright
A striking feature of the draft Code is the emphasis on protecting human creators’ IP rights. AI providers must:
Implement an internal copyright compliance policy comprising:
Upstream (development and training): undertaking a reasonable copyright due diligence before entering into a contract with a third-party data provider.
Downstream (post release): if the AI provider integrates the GPAI into its own products (is vertically integrated) guarding against ‘overfitting’ (trained too many times on the same data, which appears to contribute to the GPAI being able to reproduce copies or versions of training data). If the GPAI is supplied to downstream developers, contractually restricting those developers from breaching copyright in fine-tuning or adapting the GPAI.
Ensure data harvesting respects creators’ use of technology opt-outs (for example, Robot Exclusion Protocol or robots.txt) from the EU’s text and data mining rights. Search engines also should not disincentivise use of robots.txt by adversely affecting the findability of material carrying the opt-out.
Use reasonable measures to exclude pirated sources, such as by excluding websites listed in the Commission Counterfeit and Piracy Watch List.
Publicly release the following information, in a language broadly understood by the largest possible number of EU citizens:
Adequate information about the measures the AI provider adopts to identify and comply with creators’ rights reservations.
The name of all crawlers used for GPAI’s development including their robots.txt features.
A single point of contact to allow creators to directly and rapidly identify potential copyright breaches to AI providers.
Maintain up-to-date records about data sources used for training, testing and validation of GPAI and provide on request to the AI Office.
Regulation of models with systemic risk
What is a systemic risk?
The draft Code defines systemic risk in three dimensions: type, nature and source.
The types or ‘taxonomy’ of systemic risks are largely self-explanatory:
Cyber offensive capability.
Chemical, biological, radiological and nuclear risks.
Loss of control: inability to control powerful autonomous GPAI.
Automated use of GPAI for AI Research and Development: this could greatly increase the pace of AI development, potentially leading to unpredictable developments.
Persuasion and manipulation: such as election interference, loss of trust in the media and homogenisation or oversimplification of knowledge.
Large-scale discrimination.
The nature of systemic risk includes:
In whose hands could the risk be driven, for example, a state actor, an individual or even an autonomous AI agent.
Velocity at which the risk materialises and its visibility.
How the risk plays out: linear, recursive (feedback loops), compound and cascading (chain reactions).
Risk probability-severity ratio.
The source of the risk includes the following capabilities and propensities of a GPAI:
Autonomy, scalability and adaptability to learn new tasks.
Self-replication, self-improvement and ability to train other models.
Situational awareness.
Confabulation (generation of plausible sounding but potentially inaccurate or fabricated information).
‘Goal-pursuing’, resistance to goal modification and ’power-seeking’.
‘Colluding’ with other AI models/systems to do so.
Human intervention also needs to be considered in assessing systemic risk, such as the ease of removing guardrails.
Assessing systemic risk
AI providers must implement a Safety and Security Framework (SSF) for GPAI with systemic risks:
The AI provider must commit to “a continuous and thorough analysis of the pathways to systemic risks” of its GPAI across the whole AI supply chain.
The AI provider must use ‘best-in-case’ methodologies with ‘high scientific rigour’ to identify and assess systemic risk and must collect model-agnostic evidence about the systemic risk, including “literature reviews, competitor and open-source project analysis, forecasting of general trends (like algorithmic efficiency, compute use, energy use) and participatory methods involving civil society, academia, and other relevant stakeholders”.
For each specific systemic risk, the AI provider must map potentially dangerous model capabilities, propensities and other sources of risk, categorising each into tiers of severity.
Testing for systemic risk must include the GPAI integrated “in an AI system representative of future AI systems in which the model is intended to and reasonably foreseeably will be used, but also in an AI system where the model’s maximum potential to pose systemic risks is revealed”.
Testing of GPAI for systemic risk should not just be limited to risks or capabilities already identified, but also strive to identify new risks and emerging capabilities.
Key results from the above analysis must be validated by third party experts, especially for high tiers of severity of systemic risks.
Mitigating systemic risk
The following mitigation measures must be adopted:
If a systemic risk has been identified in the development phase, the SSF must set out the process to decide whether to proceed or not, including to spell out the pros and cons and the circumstances in which external input, including from regulators, will be sought. The AI provider must notify the AI Office prior to releasing a GPAI with systemic risk.
If a systemic risk emerges after release, the SSF must set out a process for reporting the risk, assessing its severity, decision making (including any cost benefit analysis) on responses, and any third-party verification. The AI Office is to be given equivalent access to information in this process as internal parties.
If a GPAI with systemic risk is a closed model, the SFF must specify measures to prevent and to respond to unauthorised access to weights and assets when at-rest, in-motion and in-use.
In light of the nascency of the science of AI risk management, AI providers should:
Allow independent researchers to meaningfully study the risks, limitations, and properties of models, by for example providing them with sufficient access, resources, and assurances of non-retaliation against legitimate research.
Be open with other stakeholders in AI ecosystem, including competitors, about best in practice risk detection and mitigation tools and generally their experiences with systemic risks.
It's only a draft
The EU is on a tight timetable to finalise the Code for commencement 1 May 2025.
The two key areas for feedback are:
Is there enough detail? While already at 36 pages, the drafter's query if more concrete KPIs and measures are needed and whether they have adequately captured the range of business and distribution models for GPAI (for example, there is not much mention of differentiated risks between open vs closed models).
Is it correct to assume there will only be a small number of both AI models with systemic risks and providers thereof? This assumption could be blown away with the emergence of AI personal assistant models and agent wraparounds with high degrees of autonomy.
As with much EU digital regulation, there is an EU vs. US dimension:
Another advantage for the European AI ecosystem is that the GPAI requirements in the AI Act, which the Code will detail, distribute the regulatory cost across the entire AI value chain. Companies that build AI applications using another provider’s large GPAI model as a foundation will not have to bear the entire regulatory burden. Instead, GPAI model providers, which are predominantly non-European, will also have to comply with some basic rules, and mitigate risks they are uniquely placed to address.
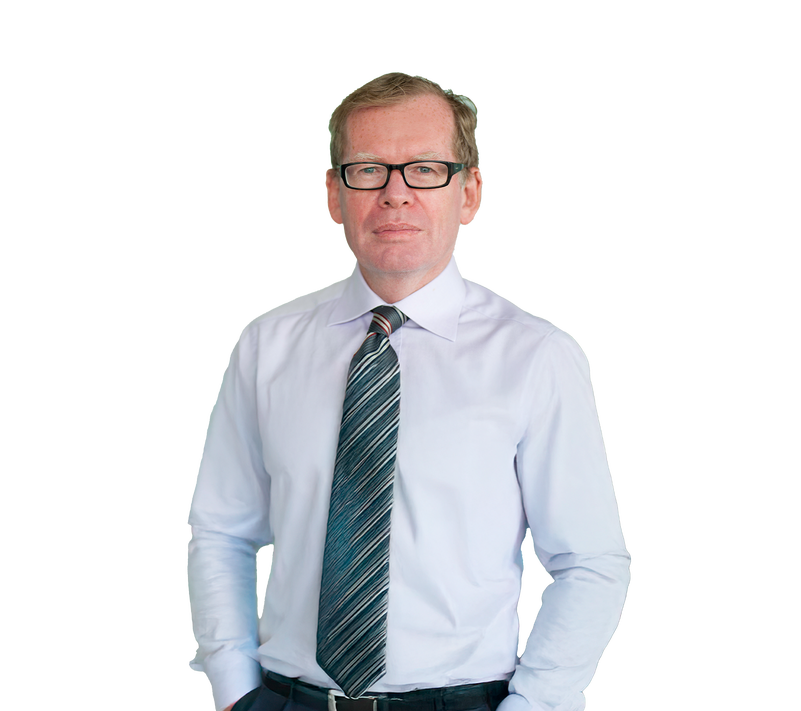
Peter Waters
Consultant