The UK Government has invested 179 million in public and private funding in Our Future Health, a major focus of which is scaling up genomic testing. Last year, the University of Melbourne and the specialist cancer hospital, the Peter MacCallum Centre, agreed to jointly establish a new centre to transform how genomics and precision oncology is delivered in Australia. See more here.
The UK’s Ada Lovelace Institute recently released a major report on the benefits and risks of AI-driven genomic techniques as predictors of people’s future health (called AIGHP).
Genomic vs genetic testing
Genomic testing assesses the collective impact of multiple (individually small) genetic variations on the likelihood of an individual exhibiting a given trait (such as developing a particular disease) relative to the rest of the population. Whereas genetic testing looks at the function and impact of specific genes, genomic testing looks at an individual’s complete set of genetic information.
Genetic testing is used to identify monogenic traits and diseases, those caused by a single genetic variation. However, most adverse health conditions and diseases are polygenic, where a person’s chances of developing them is influenced by multiple different genes.
As a result, genetic testing is of benefit in testing and treating only a narrow segment of the population, possibly less than four per cent, whereas genomic testing potentially has a reach cross the whole population.
Genomic testing can be done without AI, but is ready-made for AI, for two reasons:
Genomic testing is essentially a probability model: it does not definitively reveal whether someone has or will develop a given trait, but can reveal whether a person has a higher or lower probability of having or developing a trait.
The genomic data is one input to the predictive modelling - the other inputs are phenotype data, which captures a person’s disease symptoms, age, ethnicity, gender etc, and environmental data (called genome-wide association studies or GWAS). Powering GWAS allows vast qualities of data to be used and the discovery of complex interactions between multiple factors which human researchers could miss.
The potential benefits of AI-powered GWAS testing are obvious:
If appropriately integrated into a healthcare system, AIGHP could provide people with insight into their risk of developing particular diseases, inform beneficial lifestyle changes and help people be alert to symptoms of conditions for which they are at higher risk. At a collective level, insight into variations in disease risk across the population could inform decisions about who to prioritise for screening and help with resource allocation by providing insight into groups or areas more likely to need particular treatments.
What are the risks?
The risks of mass participation in GWAS testing are just as obvious as the benefits. As the Lovelace report says:
Insights from genomic diagnostic testing are notoriously sensitive, wrought with complex ethical challenges around a person’s - and their biological relatives’ - right to know, or not know, about a disease they may have.
AI powered GWAS escalates these risks because it could generate a huge number of different inferences about a person, and potentially those biologically linked to them, on the basis of their genomic data. Going beyond common diseases, this could include predictions about personal behaviour such as risk-taking, substance abuse, intelligence and educational attainment linked, in the ‘black box’ of AI, to some genetic components.
The Lovelace report observed there is “substantial disagreement in the scientific community concerning the levels of accuracy and utility of such systems”, for the following reasons:
The ‘garbage in/garbage out’ hex of technology: existing genome sample sizes tend to be small and worse still, most current polygenic scoring systems are trained on datasets representing people with European genetic ancestry: 83 per cent of GWAS have been conducted exclusively on cohorts of European genetic ancestry. Additionally, the phenotype data (a person’s symptoms and current condition) and environmental conditions is dependent on assessment and recording by human health professionals, which can be inconsistent, subjective and even wrong.
More fundamentally, the Lovelace report says that “genomic variations appear to account for a small proportion of disease risk”. For most common diseases, more conventional and well-established risk factors such as smoking, obesity and socioeconomic deprivation may have a greater impact than a person’s DNA. The old nature vs nurture debate also raises its head: “[m]any non-genomic influences on observable traits (such as family and socioeconomic status) have a high degree of heritability and therefore often overlap with genomic variations.” Finally, we have a poor understanding of how the genomic, personal and environmental factors interact with each other.
As a result, the Lovelace report says that AIGHP tests often can be worse predictors than more conventional diagnostic methods, such as blood tests or MRI scans. The Lovelace report concedes that AI itself may mitigate some of these problems, such as by being able to iron out the’ noise’ in input data and more robustly identify and test data patterns. But the Lovelace report also thinks it is an open question whether and when AIGHP testing will be a sufficiently reliable tool to support Tony Blair’s vision.
Guardrails
The Lovelace report recommends the following guardrails and safeguards be promptly put in place before AIGHP gets out of hand:
Privacy and surveillance
It is difficult to imagine information which is more intimate and sensitive than your DNA. Since AIGHP will likely rely on whole-genome sequencing, participation in AIGHP will probably require a person to share their entire genetic code. This would make it practically difficult for them to limit what could be inferred about them in the future.
Driven by good intentions, individuals also could face formal legal requirements or informal pressure to provide their genomic data and to consent to its largely unconstrained use, with arguments made along the following lines:
Beneficiaries of AIGHP-guided care or public health schemes have an obligation to share their genomic and personal data to help develop, maintain and improve AIGHP systems. Anyone who fails to do so is ‘free-riding’ on the contributions of others.
The Lovelace report recommended that existing privacy laws be strapped up to provide clearer, more rigorous protection of genomic data. Genomic data should be treated as personal data protected by privacy law regardless of whether the data identifies an individual (which is the usual test about whether data is protected). While there is controversy over whether current technology enables an individual to be identified from genomic data, “technological capabilities and the availability of complimentary datasets are developing rapidly and unpredictably”.
The Lovelace report was particularly scathing of current consent models. Consents are often very broadly worded and treat the giving of consent as a one-off exercise, with largely unconstrained future use by health authorities and researchers once given. The problem is further compounded by the loose approach taken to re-purposing’ data:
A [data] processor does not generally need to seek fresh consent to process a subject’s data in a new way. Instead, they can argue that the new purpose is not incompatible with the purpose for which consent was originally given.
The report conceded the need to constantly reobtain consent to use the same dataset is a common complaint of many medical researchers. To facilitate medical research, the previous UK Conservative Government had proposed to legislate a presumption that new research would be considered consistent with the original research for which the consent was obtained (i.e. onus on the data subject).
However, because of the high sensitivity of genomic data, the Lovelace report takes the opposite approach. It recommends that health and research authorities should offer patients a more granular model of consent under which the patient can specify in greater detail what they want to be done with data they share: for example, a form with standardised options that are structured to enable people to explicitly opt out of particular uses of data, including sharing data with particular entities. Then, within the framework of that tighter consent model, researchers intending to re-purpose genomic data should be required to inform the data subject of the proposed re-purposing and to seek fresh consent unless the researcher could show that the new research was consistent with the original consent (i.e. onus on the researcher).
Broad non-discrimination restricting the use of genomic test results across employment, provision of goods and services and contracting are in place in Canada, the US, and Austria.
Incremental deployment
The Lovelace report advised against proceeding with widespread deployment of AIGHP in the UK health system, at least until the technology is a lot more understood and mature. Instead, AIGHP should be introduced “carefully and deliberately to enable the targeted use of AIGHP systems for cases in which there is a well-defined need for the insight they can provide.” Even then AIGHP should only be used if the following conditions are met:
Adequate regulatory safeguards against surveillance and discrimination are introduced.
The accuracy and reliability of AIGHP systems for different demographic groups reliably reaches a certain threshold.
Adequate and timely support for those who would be subject to AIGHP insight is readily available.
The report also raises bigger, more philosophical concerns about the impact of widespread use of AIGHP on the public health system:
Given that genomic factors are the smaller part of most common adverse health conditions, would governments get ‘more bang for buck’ by prioritising “improving environmental determinants of healthcare outcomes over providing the whole population with insight into genomic variations in disease risk”?
Given the scale of computing power and data collection and processing required, would widespread AIGHP require, in effect, key steps in risk management, diagnosis and treatment being outsourced to “a handful of extremely large technology companies, whose business models are based around the accumulation of large datasets and the monetisation of insight derived from that data”?
As with medical AI generally, will “deference to clinical decision support systems could undermine the ability of medical professionals to incorporate non-machine-readable information into clinical decision making?” In other words, as AIGHP is not a binary determinant of illness, the qualitative judgment of a professional based on their experience will be just as good or will add an X factor to the AI’s more mathematical analysis.
Widespread use of AIGHP will require a massive investment in computing resources, data standardisation, staff training and the hard and soft infrastructure to support preventative health management services. But AIGHP is a gamble because it is not clear that it will result in reduced health demands - the machine predictions could be wide of the work, or perhaps more likely, the humans won’t act to head off the machine’s predictions. This could be a case of robbing Peter to pay Paul: leading to a gap between capacity to deal with acute and chronic illness and unreduced demand, thereby making the service less resilient.
Conclusion
While the technical, equity and legal concerns with AIGHP identified by the Lovelace report are very real, and the need for better safeguards is clear, its underlying concern seems to be that politicians are running ahead of the scientists and clinicians. The report’s key message may be that unrealistic expectations of AIGHP and a too-hasty pace of adoption could be driven by politicians’ desperate hope that AIGHP testing to promote preventative (and cheaper) health care will be a silver bullet for the funding crisis in the remedial health system.
Read more: Predicting The Future of Health
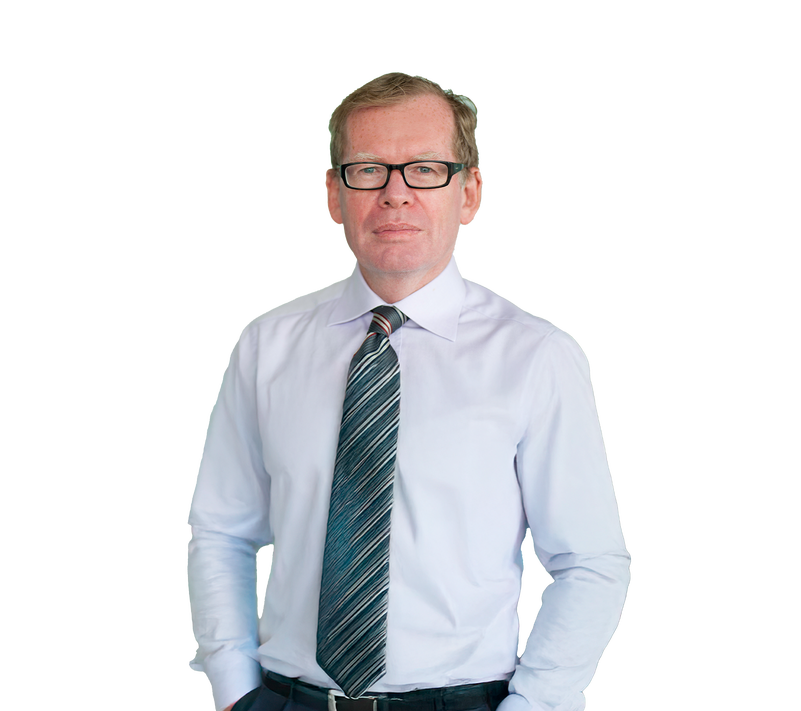
Peter Waters
Consultant